Developing Responsible AI with David Gray Widder and Dawn Nafus
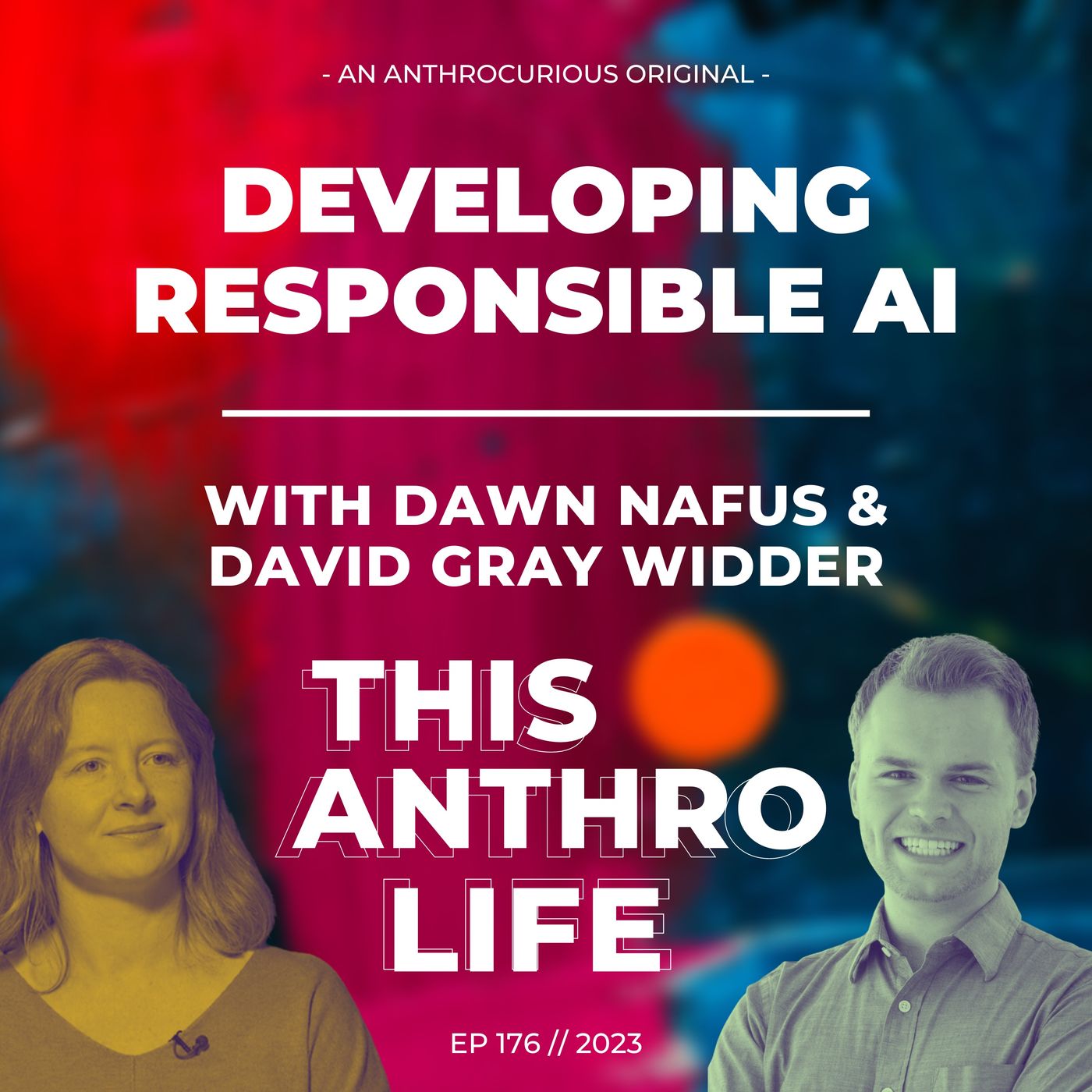
Contemporary AI systems are typically created by many different people, each working on separate parts or “modules.” This can make it difficult to determine who is responsible for considering the ethical implications of an AI system as a whole — a problem compounded by the fact that many AI engineers already don’t consider it their job to ensure the AI systems they work on are ethical.
Contemporary AI systems are typically created by many different people, each working on separate parts or “modules.” This can make it difficult to determine who is responsible for considering the ethical implications of an AI system as a whole — a problem compounded by the fact that many AI engineers already don’t consider it their job to ensure the AI systems they work on are ethical.
In their latest paper, “Dislocated Accountabilities in the AI Supply Chain: Modularity and Developers’ Notions of Responsibility,” technology ethics researcher David Gray Widder and research scientist Dawn Nafus attempt to better understand the multifaceted challenges of responsible AI development and implementation, exploring how responsible AI labor is currently divided and how it could be improved.
In this episode, David and Dawn join This Anthro Life host Adam Gamwell to talk about the AI “supply chain,” modularity in software development as both ideology and technical practice, how we might reimagine responsible AI, and more.
Show Highlights:
- [03:51] How David and Dawn found themselves in the responsible AI space
- [09:04] Where and how responsible AI emerged
- [16:25] What the typical AI development process looks like and how developers see that process
- [18:28] The problem with “supply chain” thinking
- [23:37] Why modularity is epistemological
- [26:26] The significance of modularity in the typical AI development process
- [31:26] How computer scientists’ reactions to David and Dawn’s paper underscore modularity as a dominant ideology
- [37:57] What it is about AI that makes us rethink the typical development process
- [45:32] Whether the job of asking ethical questions gets “outsourced” to or siloed in the research department
- [49:12] Some of the problems with user research nowadays
- [56:05] David and Dawn’s takeaways from writing the paper
Links and Resources:
- Dislocated Accountabilities in the AI Supply Chain: Modularity and Developers' Notions of Responsibility
- Connect with David Gray Widder via his website
- Connect with Dawn Nafus via Linkedin
- Connect with Adam Gamwell via email, LinkedIn, or Twitter
- Subscribe to the This Anthro Life newsletter
This show is part of the Spreaker Prime Network, if you are interested in advertising on this podcast, contact us at https://www.spreaker.com/show/5168968/advertisement
[00:00:00] Adam TAL: : Hello and welcome to This Anthro Life, a podcast about the little things we do as people that shape the course of humanity. I'm your host, Adam Gamwell. Today, we're gonna talk about responsible AI, artificial intelligence, and the future of the technology industry. But before we get into it, let me share a story with you. Recently, a law enforcement agency in the US deployed an AI system to predict which of its officers might need counseling based on their social media posts. The system was meant to detect indicators of mental health issues and help get struggling officers the help that they need. At first glance, it seemed like a great use of AI. But when the system was analyzed, it turned out that the vast majority of the at-risk officers were people of color or women or both, expressing their concern about systemic racism and sexism within their departments. In other words, the AI system was flagging officers who were speaking out against injustices rather than those who might need help in counseling for mental health issues. The story [00:01:00] highlights the urgent need for responsible AI development and implementation, and that's precisely what we'll be talking about today with our guests, David Gray Widder and Dawn Nafus.
[00:01:09] Adam Gamwell: David Gray Widder is a technology and ethics researcher in PhD candidate at Carnegie Mellon University. He has conducted extensive research on the intersection of ethics, technology, and labor, as well as works as a computer scientist.
[00:01:20] Adam TAL: Dawn Nafus is a senior research scientist at Intel Labs, and her work focuses on designing and studying systems that support personal informatics and data-driven decision-making, as well as exploring the ethical implications of these systems.
[00:01:35] Today, Dawn and David are gonna help us explore three questions. First: what are the limitations of the current modularity system in software development, and what impact can it have on user experience, data privacy, and end-user consumption? If you're not familiar with what a modular system is for development, no worries. We're gonna get into that in the earlier part of the episode. Second: what are the implications of a siloed approach to [00:02:00] user research and design on AI ethics and responsible development, and how might we ensure that the needs of a wider range of stakeholders are taken into account during development, including end users? And finally: what are the potential solutions to the power imbalances between labor — that's the folks that are doing the development — and management — the folks that are directing how that labor happens — and how might we build frameworks of accountability and collective action across the technology industry landscape?
[00:02:31] So this conversation is fundamentally important as AI is becoming more and more ubiquitous in our everyday lives and touching more parts of how we move about our everyday worlds. We hope that this conversation will help you better understand the complex and multifaceted challenges of responsible AI development and implementation. We're gonna be walking through some of the arguments that David and Dawn put together in a new paper. We cannot wait to dive into this with you, and so we'll jump right into it after a message from this episode's sponsor.
[00:03:08] [00:03:00] So I just wanna say, Dawn and David, super excited to have you on the podcast today. It was fun to connect with David at the Society for Applied Anthropology this year in February. And we actually just ran into each other and then got to kind of talking about the work that David was doing and immediately caught my attention as this is a conversation we need to have on the podcast and help bring to wider audiences. And I was delighted to also find that he's working with the amazing Dawn Nafus and great to have you back on the program as well. And both a very timely topic, but then also I think the work that you're both doing in terms of bringing computer science into conversation with anthropology and anthropological thinking is super important. So first off, I wanna say to you both thank you for being on the show and excited to chat with you today.
[00:03:48] Dawn Nafus:
[00:03:48] Excited to be here.
[00:03:49] David Gray Widder:
[00:03:49]
[00:03:49] Great to be here.
[00:03:50] Adam Gamwell: Right on. David tell us your superhero origin story you know, how you found your way into this space And then, we'll jump over to Dawn.
[00:03:57] Sure.
[00:03:58] David Gray Widder: So I am a [00:04:00] computer scientist by training. I come to AI from more of a technical perspective. My first class in it was thinking about like how to build neural networks and such. And in my research, I tend to interview software engineers and try and understand how they work, how they use tools, you know? So I come at this more from a technical perspective, having taken, AI courses, but also trying to understand how software engineers work, how they use tools, how they make choices, how they make decisions, how they collaborate. And that led to a few different internships, at NASA, at Microsoft and then also at Intel, where I met Dawn. And the more I learned about the existing research in responsible AI, the more I at least saw that it relied on a particular idea of what developers thought was their problem or their responsibility and what kind of power they might have to action on what they thought their job was — their sort of agency.
[00:04:54] And that then led to me conceiving of some of my dissertation research. I was thinking about, well, actually, [00:05:00] what kind of responsibility do individual developers feel over the downstream impact of what they create, and given that, what do they feel they can actually do about it? And the more I've worked across disciplinary lines such as with Dawn, the more I've tried to think about those more individual factors of responsibility and agency within sort of broader discourses and contexts that constrain and guide those
[00:05:23] Dawn Nafus:
[00:05:23] I'll pick up next. — so I've been an anthropologist in industry for an embarrassingly long time. And I turned to responsible AI back when it was really just exploding around 2017-2018, where Gender Shades had just come out. Folks were saying, "Look. This is a big deal. This is a real issue." And internally, my lab director Lama Nachman had started leading the charge about, okay, what do we need to do in-house to sort ourselves out? So we were looking [00:06:00] internally, what do we need to do? You know, Intel does put out AI models. We also have a lot of customers that use AI for various purposes. And so the question of both how do we have to govern,what kinds of models we put out in the world which is kind of where the developer experience perception understanding slash notion of supply chain became salient And where does Intel sit in all of this because, you know, we're not Google, we're not Meta, we don't really build the sorts of stuff that you commonly see in the news. So where do our responsibilities begin and end became this question that became part of my job to answer. So here I am.
[00:06:42] Adam Gamwell: Here to answer that question. And boy, folks, do we have the answer for you today. I appreciate you both sharing that, and it's a really interesting arena again to think about the importance of interdisciplinary approaches to these kinds of problems, right, because one, as we're recording this in 2023, AI is becoming more ubiquitous. I mean, the thing is it's already been kind of [00:07:00] stealthily ubiquitous in a lot of technologies. We may have used the word automation a little bit more, but now with things like, you know, ChatGPT and Open AI and Anthropic getting more press, and there's just the stupid explosion of AI chatbots on the iOS and Android app stores now that — they're becoming just more common tools on the consumer side.
What we see when we focus on software developers
[00:07:19] Adam Gamwell: And so I think what's really interesting, and I'm excited to explore with both of you today, is a lot of the work that you're looking at is on the like the production, the developer side, right? And so oftentimes, it's like how do things get shaped before they even find their way over to consumer-facing products or features?
[00:07:33] So I think it might be useful for our listeners to zoom out a little bit and describe this world that David and I have been working in, you know, at a very broad level, one of the things you'll see regularly in responsible AI reports and publications and even policies is developers should blah, blah, blah, right? They should test for bias or developers should be [00:08:00] transparent about blah, blah, blah, blah, blah. And it goes on and on. There's about a million inventories you can find online that sort of check, like, have you done X, Y, and Z? Okay. And the problem that we're poking at is we're sort of saying, "Okay, wait. Which ones?" Like, which ones do you mean exactly when you say developers should blah, blah, blah? And, that's a problem that arises in many different contexts, right?
[00:08:25] Dawn Nafus: So for example, even in policymaking right now, one of the things that many policy makers are struggling with or are trying to figure out how do you have a policy around is, for example, what happens when a model an AI model gets open-sourced, right? These discussions, happened before the generative AI kind of became hot in the news, right? Even something as simple as, does it detect a face? Like, is it a face or not? Never mind facial recognition. Just is it a face or not, right? What's the responsibility when you put this out into open source and all of a sudden, you know, anybody and [00:09:00] their brother can do this and that and the other thing with it?
[00:09:02] And so that's when we started to reframe, okay, you know, there's a notion of developers, but who developers are are actually situated in a political economy of sorts. If, you're an anthropologist, that's how you describe it. If you're a business person, you describe it as an ecosystem or you might also describe it in supply chain terms, which is what we did both because that invokes kind of corporate social responsibility but also because that's how developers themselves see the world. Like they see it in terms of a chain where they made a little part, and the little part is modularized in exactly the same way as you'd have, you know, a container on a ship, right? The language isn't coincidental at all, and other scholars have been writing about how that way of imagining the world kind of inflects what people do, right? So, we've said, "Okay, fine. You've organized yourselves." There are many different actors, both companies and individuals[00:10:00] assembled along what they see as a chain. And what we're saying is, "Okay. How do you act?" That's the social circumstance. So how do you act in that to get the results that's gonna actually work out for people. So that's why we framed that the problem in the way that we did.
[00:10:16] David Gray Widder: I think that we've seen, at least in my view, responsible AI emerge as largely a set of principles. We might have heard of, you know, fair AI, accountable AI, transparent AI, and that sort of, in a lot of company context but in a lot of like policy and regulatory context too, seems to outline the boundaries of what constitutes responsible AI. So we want to build these things in certain ways. We want to build what we're building in a fair way. Make sure the AI is fair, doesn't discriminate. But what that does in my view and some of my research has shown is it sort of scopes what people see as their responsibility, you know? If we're going to build a fair AI, we will build it in such a way that it is, [00:11:00] you know, doesn't have bias in the model perhaps. But once that leaves our hands, that's where perhaps some of our responsibility might stop, you know? The design attributes, the fair, accountable, transparent design attributes are what scope responsibility in many conceptions of responsible AI and less thinking downstream, less thinking about how the thing we build may be used. And perhaps also thinking less upstream, too. Like, you know, oh, well, our data that we're getting from the web or whatever has bias in it, but we didn't create that data, so that's not on us in some sense. And that's where we came from, you know, thinking about where I at least came from thinking about these principles and what their perhaps shortcomings or limitations are, and then begin thinking about how they might limit the kind of scope that individual developers told to and believing certainly that responsible AI is important then may find the limits or the scope of what they see their job as being. And that's then where the AI supply [00:12:00] chain or value chain sort of notion became helpful and that it sort of helped us zoom out from one particular developer's context or sense of responsibility to seeing how they relate to the many other developers, the many other modules that are necessary to create finished AI systems and how responsibility does or does not sort of transfer throughout those chains.
[00:12:22] Adam Gamwell: Gamwell: I think that's a really important framing you're inquiring about this because there is, of course, the literal business and lived reality of how developers work in a system, right? and I think of echoes, too, of — I mean, this sounds weird to say this but like assembly line factory labor, too, right? That you have your part of the car in Henry Ford's factory, you build this part of the car, and you do that over and over again. And so you're not responsible for when the car gets on the road or are you? is one of the questions that y'all are poking at.
[00:12:47] And I think, you know, you're absolutely right there, too, Dawn, where it's the language that we use shapes how we approachthe process of what we think about what it is that we're, quote unquote, supposed to be doing. And so the kind of question I was [00:13:00] opening us up with to is like responsible AI as again a set of practices as like where did it come from versus a sense of responsibility that an individual has. And then, I think it's really important that you've exploded that to say we have to look between these two in terms of if we're talking about developers should do something which developers, who's making it and where, David to kind of draw on some of the language you too, in terms of are we upstream, are we downstream? Where are we in the supply chain? Are we very close to consumer end products and features? Are we at the first base code, right, where a consumer will never see that unless they're some GitHub nerd that wants to dive into it if it becomes open source later, right?
[00:13:36] And so one thing I'm curious to think about, too, is this kind of typical process. You know, Dawn, you began walking us into this space. And so thinking about what does that development process typically look like? So we have the both the metaphor and I think the reality, right, of working in these modules, right? These like these sections of ship as it were that they kind of help move part one to part two to part three to part four. But then also, I think the other piece that I wanna hear from you two is like how developers also [00:14:00] see themselves within these modules. So David, from your perspective, how are developers thinking about themselves in the development process as part of this chain as part of these different modules? What does that look like for them?
What is Modularity in Software Development? Why has it been so hard to question?
[00:14:10]
[00:14:10] David Gray Widder: starting with something you sort of said a little bit ago, thinking about modularity I mean, I sit in a PhD in software engineering program where we read sort of the papers that said, hey, one day systems are gonna become so big we can't, think about them all at once. We have to think about modularity, and this will enable all these wonderful benefits such as division of labor in the factory context you just talked. And so really, you know, modularity is held up in software engineering as sort of an unquestioned core principle that allows us to build, to scale, to build large systems and have someone be responsible for one thing and then someone else be responsible for using that thing but not knowing how it works.
[00:14:50] So in terms of, how does AI finished AI systems today get built, modularity allows it so that not each developer has to understand the inner workings of the whole system [00:15:00] so that we can, for example oh, this is the standard data set. I got it off the shelf. I don't know exactly how it was collected and I don't know exactly, you know, the issues at play, but we agree that this is the standard module for this kind of thing. Or this is the facial recognition model that we're going to use and really don't need to know the minute details of how it works, but we can tune it to work in this particular context or whatever for this particular product.
[00:15:25] So, none of this is terribly controversial. I mean, people use these sort of kickstarts to make software engineering and building AI systems faster to make it so they can be built larger without having to intimately understand the inner workings. And developers sort of see this as part of being a good developer. You ought not make the person using your module understand too much about how you built the thing 'cause it ought to work and just, you know, render its interface easily understandable to the next person.
[00:15:53] Dawn Nafus: You know, as Dave is talking — well, I'll say two things. First is that I think doing this work with David helped me [00:16:00] appreciate that there are times and places where it actually does make sense to not have to get into the inner workings of every single little thing, particularly when you're building complex systems. I mean, I think I've come to appreciate that more. At the same time, it has all the hallmarks of what Charles Perrow would call normal accidents, right? You build up a system that is sufficiently complex where no one person necessarily, understands the whole because you can't. Cognitively, you just can't. That's a pretty good scenario for opening the door to failure, right, because you have systems that layer upon systems, upon systems, and then all of a sudden in that layer and you have a through line to badness happening, right, of some kind. His example is nuclear power accidents, right, why they continue to happen despite all the [00:17:00] safeguards thrown on top and thrown on top and thrown on top, right?
[00:17:03] In this context we have something similar where everything things do layer over time and it is actual work. I mean, part of the problem here is it takes work to actually go digging through, oh, wait. Is the problem actually on the training data side, right, what the models take in and learn from? Is it in — oh, actually something went wrong with the model itself. Like they didn't tune it in the right way. Is it in weight, the entity, the company putting it out in the world actually, could have made better choices about what context? Or is it the end use, right? one of the things we know from the anthropology is of course using technology is a creative endeavor, right? That's just true. So, so there's this distance that you've gotta travel through and it takes a lot. It takes both a lot of work and a lot of kind of intellectual agility to go through all of that difference, right? you know, Modularity's there for a reason.
[00:17:57] And really what we're calling [00:18:00] for is an apparatus to, to help folks traverse through it, right, so it might be that, instead of just saying, look, okay, here's my box. I did my work, right? Maybe it's things like asking the person whom you received your parts, right, your data, whatever it is, or your open source model, "What's in this box?" Like what do I need to know about this box, not necessarily what's in it, but what do I need to know about how it behaves, right, and building that reflex a bit better, right? Like things like that that kind of deepening the connections are sort of the thing we're after here.
[00:18:32] David Gray Widder: And not necessarily just like what do I need to know what's in this box to use it, which is kind of the state of affairs now. But like for purposes of thinking about ethical questions, like what do I need to know about what's in this box for thinking about my own relation to the next person who might use what I'm building and, taking away, oh, this is a standard component as sort of a common refrain and thinking more, no, like I'm building a system that is using a standard component, but I still want what I'm building [00:19:00] to not cause harm down for the next user or for the people who deploy it downstream or on whom it's deployed downstream, you know? I want to think more about like what I'm depending on, you know, who I'm depending on and what the not just will it work, will it fulfill the immediate goal of what I'm trying to build, but which is already fulfilled through modularity and sort of the interfaces that permit functionality. But thinking more about asking the same kind of questions that Dawn outlined for ethical reasons.
[00:19:27] Adam Gamwell: Adam As like an analog, if you're writing a dissertation or a research article you are in essence doing something similar of pulling, a library sometimes literally of previous research and ideas and thinkers to then amalgam them in a different way that can then be consumed in a new way for different audiences. And we may not think about — maybe we should — causing harm the same way when we're putting a paper or dissertation or book out into the world. But, I mean, if we are putting something that people can consume, obviously a book doesn't make decisions or maybe shape the way a traffic light works the way that generative AI may at some point. [00:20:00] But the modularity example I think is really interesting. then I do think that there's good ways to echo that in a typical research process, too.
[00:20:06] And just again so if folks either don't work in computer science or like, it still feels like a black box, then part of it is this, too: like what resources are we drawing from ahead of time to then build into our system now to then see where it goes next. I know we may find ourselves at one point when a book itself can also then, you know, write its next chapter. But that's — I think we're not quite there yet. I mean, maybe we are.
[00:20:27] Not any chapter I'd wanna read.
Modularity shapes what - and how - Developers Know their Work
[00:20:30] Dawn Nafus: Exactly. You know, it's funny you mentioned writing, Adam. I mean, I think one of the things I learned in writing with a computer science student is that like modularity is really epistemological. It's not just "I know what my job is and I do my job." It's like it gets into how you think to the point where computer scientists actually don't use word processors. This is a thing. It's called Overleaf LaTeX or [00:21:00] LaTeX. I've heard all the, you know, many different ways of pronouncing it. And it's wild because — okay, it's wild if you're an anthropologist, right? It's totally normal if you're a computer scientist. But I am an anthropologist, so I'm gonna call it wild. And what happens is like the way you write is you code and then you render, right? So it's lines of — I mean, it's lines of text. It's legible. There are a couple commands that if you're smart, you could learn potentially. I did not. But, other than that it's like the final text is a separate thing. It's like, you know, compiling code. And it's meant to be like code is meant to be built in modules like — and apparently, text in computer science is also meant to be in clean modules, and I couldn't do it. I could not write this paper like 'cause there were these parts and there was no — there were no visual assets to actually build an interlocking argument in a [00:22:00] way that I normally would and it drove me bonkers. David, I'm sure is very confident, you know, happy with LaTeX. But I couldn't do it.
[00:22:08] David
[00:22:08] David Gray Widder: Widder: Well, again it's the unquestioned norm in my field. Like I also had to learn this early on in my PhD and, you know, didn't have fun either. But like to collaborate with anyone in at least within computer science is sort of expected. to go back to the point, you know, Dawn was making, like, yeah, this is designed to with particular like rational goals in mind. Like it's supposed to save labor and that you can literally import package, use package. Like, oh, you want a package that's going to make really fancy tables and format them nicely, so you ideally can save labor at least if you know how to use them. You import a package. You import someone else's fancy table module, and then use it. But once something goes wrong with one of those packages, good luck trying to fix the problem. Or also then once you try and sort of render the thing or work with people who are used to what computer scientists would call WYSIWYG, what you see is what you [00:23:00] get editors like Word that's where problems and/or hilarity ensues.
[00:23:04] Modularity is sort of seen as a technical practice for people in my field. It's seen as just simply the way we build code and we have debates about the right way of modularizing things, you know, to fulfill one value or one goal or another. But one of what Dawn correctly pointed out as she spoke there was it functions more as an epistemic culture. It sort of transcends a purely technical purpose and then begins to be used as sort the way we think or the way we organize different concerns, separate concerns to use sort of the lingo in our field. And the hardest thing about trying to explain the point of this paper to people within my field is drawing the connection between modularity as a technical practice, which we've talked about the implications and benefits and disadvantages of here, and also explain how that sort of then transcends and becomes an [00:24:00] epistemic culture where it's seen as the right way to organize relations or the right way to do work, or the right way to do much broader things, And so as I try and explain the implications of modularity, it's hard to get people who see it as a technical practice and purely that to see some of its perhaps what we might call like ethical side effects or implications in the way we think as computer scientists.
[00:24:24] Dawn Nafus: yeah, at the end of the paper, we have these three different proposals, right? So everybody recognizes that this is a real problem. You know, what do you do about it? And one of the things we say is, look, what you do about it depends precisely on how committed you are to modularity in the first place, And so, if you are like this is your bread and butter, this is what you do, right, like the action you take is even better modularity that solves the problem, right? So you assign somebody to do the bias testing, [00:25:00] right? you frogmarch your user experience people into anticipating the usages so you know, and then you can define like what the right usages should be going forward and what's outta scope, right? Even more, you just divide the modules according to meeting the requirements of the chain, right?
[00:25:20] And then, way the other side, we essentially had a kind of a just reject the notion of modularity entirely, right? So there are examples of this where if we look at Mary Gray's work, we look at what the folks over at DAIR are doing, which is a research institute recently set up after Google got rid of it, many of its ethicists, right?
[00:25:42] What they're doing is they're saying we do deep community work, right? We take our time. We make people as much of a part of the development process so much so that it doesn't matter what technology we end up with or not. The point is to meet people's needs. Period. Right? This is not a [00:26:00] technology project. This is a problem-solving project. And if we have the technical skills that actually help, great. If not, also great. We're gonna solve the problem.
[00:26:08] And then, in between, we have a notion that takes more advantage of the institutional forms that businesses often use, right? Might be contracts, a bunch of other stuff that is at neither end.
[00:26:18] The amazing part was the people who read this paper who come from David's part of the world were like, you can't reject modularity. Like how would that even work? Like you can't build anything, right? That's just not even possible.
[00:26:32] But then on the other side we had STS (Science and Technology Studies) reviewers saying, — the implication was I know you're just putting in this improved modularity thing as like this giveaway, but really the point is to reject it obviously because the whole point of the paper's leading up to rejecting modularity, right?
[00:26:48] Adam
[00:26:49] Adam Gamwell: Gamwell: You're just softballing it in the middle, right? Yeah, yeah.
[00:26:50] Yeah, we're
[00:26:51] Dawn Nafus: just softballing it because I'm gonna come from industry, you know, like you know those people, you know? So I was like, wow. Like [00:27:00] entirely different points folks got from the paper. Was wild.
[00:27:03]
[00:27:03] Adam Gamwell: Is that a function of the fact that anthropologists and computer scientists are approaching this together — that there are these multiple interpretations?
[00:27:10] David Gray Widder: I think that, the responses like I mentioned that I've gotten from computer scientists sort of speak to why I think it's important to think about how we might improve ethics within dominant logics of modularity that exists today. I mean, like, it's both funny that the first question people tend to ask me in computer science when I talk about this paper is, oh, well, how can we modularize ethics? Like what are the interfaces we can, reveal from our modules that will, like can you do an API call for bias or you know, like people will start trying to then see it as a modularized or modularizable problem, essentially presenting modularity as a dominant ideology that sort of subsumes and organizes other concerns like ethics beneath it. At the same time , modular systems are what structure large systems today. And other people in [00:28:00] my field will ask, well, like where does it end? Like if we can't depend on modules, can we not depend on compilers? So modularity is designed to manage complexity. How else would we build large systems? Now, again, that reveals sort of an idea that, you know, we ought to build large systems and that's sort of the natural goal we must do or the natural goal we must hold. So, you know, a practical critique there.
[00:28:22] But and the more I think about it, the more like I think this speaks to sort of a different orientation from at least computer science and anthropology, which is like computer science tends to see the value in building new things like building systems. And I actually had someone ask me recently, like, how long did you have to build things before you were allowed to just comment, you know? Reading this article or — and, of course, like they were joking with me. They're a friend, you know? But the first goal of this kind of work, of this paper at least, is not to say this is how we [00:29:00] might build things better. The tagline of my department that's printed on our T-shirts is build it better. So that sort of shows you something about the orientation of software engineering departments perhaps. Whereas thinking more carefully about sort of the epistemological questions embedded in the way we build things is seen as much more in anthropology at least, you know, Dawn can speak more to that, but I see that as more what I've learned from working with Dawn, what I've learned from collaborating with an anthropologist here. But like the common refrain of like, "Well, you pointed out a bunch of problems. Now what?" spurred us to think about the menu of options approach, like, okay, so you'll have some readers that are very much wanting to know what they can do better within the ethos of modularity. But then, of course, we'll have other readers who are like not necessarily wedded to or sort of schooled in modularity who want to imagine other different futures that might have no immediately applicable within many organizational contexts sort of practical ability to be implemented.
[00:30:00] Dawn Nafus: [00:30:00]
[00:30:00] Well, I I would not call Mary Gray's project not practical to implement. It clearly was.
[00:30:06] David Gray Widder: Well, within certain organizational structures, yeah. Like that's all I meant. You know,
[00:30:10] Dawn Nafus: all props to Microsoft, right? It did happen. You know, she is at Microsoft. So there are times and places where you can do that, certainly nonprofits NGOs, foundations, right? I mean, there's a whole world out there that with proper science policy could actually proliferate and could actually really develop and elaborate the kinds of, you know, broader, social and technological trajectories that we might wanna have, right? That's not the world we live in now because we have, an unequal situation. But I think they're entirely practical that side of things.
[00:30:48] David Gray Widder: Yeah. And we get to that a little bit towards the end when we talk about the idea of like, there's this unquestioned idea of like general purpose modules. Like, well, general purpose for who? Like the standard bricks or the standard shipping containers or modules that you [00:31:00] can take off the shelf to do certain things. Well, do the existing general purpose modules exist to serve all of the kinds of things we might use software for equally? Or do they tend to push towards particular ways of using software for particular people, for particular needs business needs? So like, what would, you know, I don't know. As Dawn mentioned, perhaps like public funding for software maintenance and creation and sort of thinking of it as a public good approach perhaps or, you know, implications for policy in that direction.
[00:31:32] Adam TAL:
[00:31:32] We're gonna take a quick break. Just wanted to let you know that we're running ads to support the show now. We'll be right back.
Is there something about AI that sparks these conversations
[00:31:42] Adam TAL: I is there something about AI in general that sparks this kind of conversation of what is my responsibility? If I back up 10 years and I'm developing the code for Facebook's social graph, we think about that a bit differently in terms of a knowledge graph, [00:32:00] even if I'm, I don't know, putting my notes together in obsidian or something, right? Like is it something about AI that that makes us say we actually need this pause. I mean, obviously, there's been, you know, Dawn you mentioned problems at Google with the firing of Timnit Gebru and other issues like silencing or firing ethicists for raising questions.
[00:32:14] And I'm just thinking out loud here wondering both from reading your paper and through our conversation and just other things that we've seen recently, Sam Altman, the CEO of OpenAI, was testifying before Congress, I was also perusing Lex Fridman's podcast and he had Max Tegmark who was talking about the case for halting AI development or at least just slowing it down, stop being in a, quote unquote, arms race to be the first out of the gate because GPT-4 is actually just the baby program.
[00:32:38] Adam Gamwell: We think it's a very pretty baby, but it's the first iteration of many more complex things that are to come. And so this begs the bigger question, how will AI change society?
[00:32:47] Or are we just more conscious now that we have social media, that's changed society in many ways -mostly for worse, some for better- that we're thinking more intentionally about the impact AI might [00:33:00] have.
[00:33:00] In other words, is there something about AI that begs us to rethink the development process? Such as modularity. It seems that there's a sense of urgency from the examples that we're talking through, that AI will get out of control at some point, if we don't put safeguards in place. And so I'm curious from your perspective, how is AI reshaping, how we approach the problem or process of development?
[00:33:22]
[00:33:22] Dawn Nafus: You know, the issue is always what counts as AI is a moving kind of goalpost. And, you know, when you get into the weeds of it, as far as I understand it, very few people actually use the term AI although recently it's become more popular as it becomes the term in the popular press. And I think you're right to point out that, if you look at technologies and harm, right, there's a long list of them right through the ethnographic and sociological record that we could point to and arguably responsibility for that should have taken been taken well before machine learning came on the scene, right?[00:34:00]
[00:34:00] I would love to for somebody to actually dig into this properly. My guess is that, when something like Gender Shades comes up where it's so palpably odious that you have somebody not being seen literally because of who they are, you can no longer deny say that, oh, tech is neutral and the only problem is how you use it, right? Because clearly not, right? So that might have been part of why we're here.
[00:34:32] I will say that I think the current sort of elaborations of the moment where folks have varying reasons to be concerned about generative AI, shall we say, personally, as a, an anthropologist, I would say that some of them are more realistic than others. What I am concerned about, again, as an individual is being distracted by made-up harms at [00:35:00] the expense of, you know, the actual harms that really exist. And not only that, but for the flavors of machine learning that aren't generative, are we gonna suddenly give up because now we're worried about something that puts speech together? All of a sudden, we're anthropomorphizing, putting an imagination of personhood where it arguably doesn't belong, and so that's just fueling the fire, I think.
[00:35:22] David Gray Widder: Also, I have friends who are, getting PhDs in more of the technical aspects behind AI, and there's a huge disconnect with between what they do and what like most systems marketed as AI are or are doing. I think like almost any software is now labeled AI software, at least in the public way it's messaged or the way it's marketed. And yeah, I think that can be distracting and sort of lead towards conceiving of AI ethics and questions of superhuman possibilities, like sort of science fictiony scenarios like Terminator and,[00:36:00] the AI that might kill us or might, you know, AGI is what I'm drawing what I'm going towards here — artificial general intelligence- singularity.
[00:36:08] And with that is the very life-like ChatGPT, sort of human-appearing, convincingly putting together strings of words that sound very much like a human. But when you actually have domain expertise and what it's bloviating about are less than.
Does focusing on Responsible AI render ethical use out of scope for developers?
[00:36:23] David Gray Widder: But also in some of my more recent work, I've sort of begun to question the impact of the conceptual category of AI in responsible AI discussions. Because as we talked about earlier, like there's a certain kind of question that's like, how do we build this thing ethically? And there's another kind of question that's like, how do we use this, or how will we permit this system to be used ethically? in what ways can we sell the system? In what ways and who should we sell the system to? And scoping the question to specifically responsible AI, scopes the question, at least in my view, to a design question. Like, [00:37:00] okay. Well, we're using AI to do this thing. Now, we figure out how to design it ethically. And now, we figure out how to build it in an ethical way. Like we want to be fair, we want to be accountable, transparent, whatever, right? Now, that to me is another kind of trick that distracts us from, well, the question of whether or not we need to use AI in the first place — machine learning or statistical techniques — in the first place to do this thing. But then also, renders out of scope some of the more difficult to answer questions when you're an individual, a software developer in a business. And this speaks to our study, too. Do I want my software being used by the military? Do I want my software being used in this way for this purpose that I might have concerns with?
[00:37:41] So AI in itself can scope the kind of questions in a more narrow sense to questions of design rather to questions of use. And that might be something we want to rethink as we think about AI ethics 'cause AI is certainly the term of the moment and the term of art used in both business and in a lot [00:38:00] of development context, too. But I think a lot of the same questions can be asked about software ethics or about technology ethics more broadly. And the and not doing so can have certain implications for what is legitimate to discuss
[00:38:14] Adam Gamwell: or not.
[00:38:14] That's an incredibly helpful framework to think with because as a outsider to software development, you know, as I think about what we're talking through here, there is this question of the role that AI is also helping us then reflect back on, to your point, David, like the broader elements of software development in the first place, right?Like how is something that's like hot on people's minds, helping us reflect back on the entire production process? I think it's really valuable to ask this idea of, if we're falling into a moment of we're trying to design something, we then we might miss or scope out the question of ethics because we're not talking about its use case.
The danger of equating your user with your (desired) technological trajectory
[00:38:49] Adam Gamwell: As someone who has taught in a design program, taught design thinking methodologies and research, I'm thinking about this because teaching UX classes, user experience classes know, we always talk about, remember one of your first things of doing user research[00:39:00] is to find out who it is that you're designing for and when we are talking about design versus research, right, is there a level of research or user research that kind of goes into how we might think about this, too, of either how quickly or at what point ethical questions do get asked? Like do they tend to get outsourced to the research department, I know in the paper you noticed in the development chain, it's like they tend to get pushed into different model cards later. But is the research team also an area that we tend to see the ethics questions get siloed or moved over?
[00:39:27] Dawn Nafus:
[00:39:27] Yeah. It's like twofold, right? So, the often user experience research comes in at the end of the chain and there's an actual interface, there's an actual product. Like the at that level then, that's when the user experience folks typically have something to say. I think it's also the case that it's useful to do research kind of at that innovation level, which is one of the reasons I do stay at Intel for as long as I do is that that is what we do, you know? We do [00:40:00] include social research in the process of doing AI innovation itself, specifically to set trajectories in a way that are going to have social utility and, you know, a sense of responsibility from the start, right? That's something a big company can do that arguably is harder for smaller companies.
[00:40:22] What I am concerned about is, being a nerd and understanding other people's nerdiness, and at some level I do get this, oh, like, chasing the technological curiosity. Like, oh, wait. If I could just make it do this, you know? Like but then, you end up in a situation where your user is like the technological trajectory itself, and that's why we're here, right? Right? I mean, we have people publicly making up uses on behalf of new forms of AI for no good [00:41:00] reason, right? I've seen statements to the effect of generative AI, of course, will positively impact X, Y, Z, but there are negative implications. It's like, have you demonstrated that? No. Like you're just saying things now. Like, so there's a lot of just saying stuff. And arguably, more research is necessary. But then, you get that flip side of, when research as a whole enterprise, right, universities, think tanks, all the rest of it are sitting around chasing the trajectory that's essentially been set by, a combination of very powerful, usually white men. And, you know, the way that the engineering's unfolding, right, then, like at some certain level, those wise minds might be better spent looking at other more socially productive things, right?
[00:41:55] So we're in this kind of vicious loop of chasing the things that, you know, [00:42:00] really need significant levels of control at the expense of things that could potentially be better.
[00:42:07] Adam Gamwell: That's a great point. That how are different people's expertise like have to be deployed, right? Like we have finite amounts of time and energy, and so if we're chasing these rabbit holes sometimes — sometimes legit threats, sometimes not, right, you know, then it does take away from these other projects that have incredible value. That's a really interesting question, too. Yeah.
[00:42:24]
[00:42:24] There are a few points that Dawn excellently laid down there that I just wanna pick up on. One we make in the paper, which is the idea that like, user research leaves out actually a lot of people nowadays 'cause software systems are deployed on people who are not necessarily users in the sense we think about them. There are people who are subject to algorithmic systems are rarely the people that were sort of seen as the paid user. And so when we frame it as a user research problem, we're probably thinking about our customers or probably thinking about a particular frame of person who willfully engages with the system or [00:43:00] at least knowingly engages with the system rather than the much more pervasive effects that happen or that can happen for people who aren't in the user role. You can think about like maybe the police department are the users of the predicted policing software, but the data subjects are the ones whose thoughtcrime is being predicted or future crime is being predicted, right? Those are data subjects and those are not at least with what I've been exposed to in my classes and some of my work is yeah, users are thought of as one way. And we're hoping to think about the broader stakeholders, the broader set of people implicated in how systems are deployed and developed, and along with the broad many more other researchers focusing on that question specifically.
[00:43:42] David Gray Widder: I also think like when we, you know, is this a user research problem? I mean, I think this quite rightly, as you pointed speaks to what is valued by different sort of roles, different sort of titles within orgs. Like software engineering has a particular history and a particular [00:44:00] culture coming from that history of like what is valued as a software engineer, like making working code, making it faster, making it more efficient, making it more modular. There's various like ways that people have written about this, but code is good things and we talk about in the paper how I think it was a technical lead was glad that they were hoping to soon modularize out the parts where they had to deal with people. We have sales leads to do that. We have user experience researchers do that. So sort of a conception of what is within scope for particular role or not can make it harder to then reassemble the various sort of partial knowledges that are necessary, the deep technical knowledges that are necessary to understand the tech, you know, the implications of the technical choice on the wider world, and then the concerns about the wider world that are then implicated or that have implications for how we design the technical thing. So that's part of why we frame sort of the paper as, well, if we have dislocated accountabilities, how can we actually reassemble partial knowledges using the broader frame of the supply chain or the value [00:45:00] chain?
[00:45:00] And on the point of ChatGPT and all that and we see this like modularization of concerns — it's not my problem or concerns — and the way that has been talked about. Like, maybe some folks at least listening are familiar with the Sparks of AGI paper, the paper that said that ChatGPT is demonstrating sparks of artificial intelligence and because it can draw a unicorn well or something. But one of the claims they made in the paper is that since we don't have access to the vast full details of GPT-4's training data, we have to assume it's seen every benchmark. So the in this sense it's sort of saying, well, it's probably seen everything on the web. And so in carefully inspecting, you know, its data set to make sure that it is appropriate for use or doesn't have bias. And then, as Dawn said, disconnected from any sense of what in particular it will be used for, for whom it will be used, what problem is it trying to solve, we see that disavowal as like the data set is out there. The data is not our problem. We are building [00:46:00] this thing.
[00:46:00] Adam
[00:46:00] Adam Gamwell: I think it's a super valuable point across both of what you're saying that it's easy, I mean, upon reflection to see where and why some of those disconnects can take place, right, in terms of what is my responsibility, what's the scope of what I'm producing and making based on my expertise of technical knowledge, you know, that's either a user problem or it's that I can outsource the question of like, how will it be used?
[00:46:20] you're both approaching this I think this really important and challenging question that is taking stock of the existing epistemological framework of how developers do what they do, like how we make software today, right? We use modular systems in order to understand I'm taking part A; part B, I'm gonna borrow from this library. I'm gonna hand my box off to David. He's gonna put his pieces in there, do his thing to hand it off to Dawn, and blah, blah, blah. It's gonna go down the chain. At some point, some users are gonna try it out. But the police example you said was actually really great. Like , when you say users, we're actually like the police may be the users of the technology, but actually the subjects of the data are an entirely different group of people that have no input or, you know, they probably didn't give permission to be recorded in the ways that they were recorded, right, and what happens with their data. So [00:47:00] this is, I think, one of the big challenges.
[00:47:02] And also I appreciate why you kind of present three menu options, right, of like, what could we do with this system in terms of do we just burn it and get rid of modularity? Do we like actually increase relationships between them, you know? What is kind of the way of finding our path forward? So I think just, I'm kind of coming away from this feeling I don't know if more optimistic, but at least that the contours of the problem feel clearer. So asking about that, you know, what do we need to be paying attention to, you know, going forward what's like caught your attention because of doing this paper? Like, what do you now see that you didn't see before? :
[00:47:32] Dawn Nafus: you know, I appreciate you pointing out that, part of the job of the researcher is to identify the contours of the problem. And I will say that in the course of drafting it, putting out preprint, finally putting in proper publication that just, through time, the acknowledgement of that this is a real issue is coming from a lot more quarters, than I think it did when we started. So in that sense, [00:48:00] the, on the optimistic side, that is what I would be looking for is people really digging into, what are the relationships between different institutions, between different developers. If I had a crystal ball, there might be an elaboration of what developers already do today, right? So as an example, we found, we found cases where developers would slow-walk dodgy work. They would find other projects to prioritize because there's always something else to prioritize, right? So we might see more activity there. I mean, certainly, as regulation gets more serious, right, the game changes again, and that seems set to happen. So it's not clear. And then, you know, we'll also have to see what the next flavor of the day is.
[00:48:44] Adam
[00:48:44] Adam Gamwell: Gamwell: Also true, yes. That is right, yes.
[00:48:46] Dawn Nafus:
[00:48:46] But it always inflect old issues, right? I mean, you know, we've always had, you know, supposed AGI, right? Like that's been a long-term thing, so it'll surface things that are old. That's set to happen.
[00:48:59] Adam
[00:48:59] Adam Gamwell: Gamwell:[00:49:00] just to jump down that real quick. That's exactly right, and this is where it's even like AI feels new, but it's making us rethink the existing way that we make software, right? So it wasn't like, oh, this is new how we make software. It's more like, wait. Let's think about why we're making it the way that we're making it because there's new implications for it. So I think that that's a great way to think about that.
[00:49:16] David Gray Widder: since Dawn and I did this work, I've done some follow-up pieces that ask a question like, you know, okay, so say we have developers who want to ask the down-the-supply-chain questions, who ask like, well, hang on. I'm uncomfortable that my software is being used down the chain by the US military. what we did in that in the sort of follow-on work is survey a bunch of software developers with ethical concerns. And when they try and raise those concerns or they try and act on those concerns, I mean, they don't really have power to. Power is modularized itself. People have power over a particular part of the supply chain. So I think that, if I had to go from that to sort of thinking about the [00:50:00] future, we're gonna think about different ways that we can build power either in different parts of the supply chain through collective action, through sort of organizing efforts, or we're gonna think about ways that we can build power. I mean, and part of that will be building power sort of by connecting different parts of the supply chain, like having more relationships outside of the chain that allow people to control more of it or to understand at least the impact of what they're creating down the chain.
[00:50:29] Now, also, I'm thinking about again, how we conceive of what our job is, how we conceive of what we're allowed to raise as concerns, and sort of the scope of those. So in some very recent follow-on work is thinking about different ways that we can give people sort of social permission to think or think in hypotheticals that don't speak to what they're currently building and allow people to talk about concerns or ethical concerns that they have in [00:51:00] ways that aren't a part of their ordinary day-to-day assigned duties or job roles.
[00:51:06] I mean, if I had to sort of outline where I think we should go next, it's really starting to interrogate questions of power and asking whether this is a, you know, in a lot of framings of AI ethics, just a question of individual developers building software better or is there more collective action and collective power that needs to be built? And then also thinking about opportunities for action that we have within our own roles to say and think about things that aren't necessarily within our particular job module or job role.
[00:51:35] Dawn Nafus: And I'll just very briefly add. If the future involves more computer scientists using phrases like "partial knowledges" in a sentence, I will also consider to be a better future. More computer scientists talking about the how power actually works, the better off we are. Gamwell:
[00:51:55] Adam Gamwell: Sign me up, too. That sounds right on, you know? It's like let's get some meta modules going [00:52:00] aside to the other modules or something, but just wanna say, Dawn and David, thank you so much for joining me on the pod today. This has been an absolutely fascinating conversation, and I'm really excited to dive in with our listeners too and just get a sense of like where folks are at with this 'cause, I mean, AI is loud as we know, right? It's everywhere in the news, pulling us away from our desks constantly to go look at some other random story. And I think that the work that you're doing here is really important of helping us actually take a moment to shine the light let's actually reflect on the process of how this gets made in the real world with real developers and ask is that process, this epistemological technical process, the best way forward if we're thinking about a broader scope of, you know, well-being and a bigger sense of users — a bigger sense of implications in ethics and roles. So it's a big order, but y'all squeezed it into like a 16-page paper. So that's an excellent start and excited to see where we can build next. So thanks so much for joining me on the pod today.
[00:52:51] Thank
[00:52:51] David Gray Widder: you.
[00:52:52] Wonderful to be with you, Adam.
[00:52:54] Adam
[00:52:55] Adam Gamwell: Gamwell: And that's a wrap for this episode of This Anthro Life. We've had a fascinating conversation with our [00:53:00] guests today about responsible AI development and the role of developers in creating ethical and beneficial software products. I wanna extend a huge thank you to our guests today, David Gray Widder and Dawn Nafus. Thanks again for sharing your insights and expertise with us.
[00:53:13] Now, let's recap our top three takeaways from today's episode. First: modularity in software development is a double-edged sword. It allows for a more efficient creation and deployment of technical systems but potentially limits user experience and data privacy; second: user research and design must consider the broader stakeholders and people implicated in the deployment and development of systems beyond just the paying customer or knowingly engaged users; and third: dislocated accountabilities require the reassembly of partial knowledge using a broader supply chain or value chain framework.
[00:53:51] And as always, I want to hear from you. Do you think it's possible to give developers more power to act on ethical concerns within their organizations, and how could [00:54:00] collective action and connection between different parts of a supply chain play a role in this? Or what steps could we take to ensure that the broader stakeholders and people implicated in the deployment and development of algorithmic systems are considered in research, design, and development efforts rather than just paying customers or known users? And as individuals, what actions can we take to ensure responsible AI development? Can we also work collectively to address these challenges around AI bias and accountability? Remember you can get in touch with me over at thisanthrolife.org on the contact page or reply to emails if you're on our newsletter list. And if you don't get emails from us, I highly recommend you subscribe over at the This Anthro Life Substack to get newsletters, blogs, and to be up to date with all the happenings in the Anthrocurious community.
[00:54:46] And lastly, I want to ask you, my dear listeners, to help spread the word about This Anthro Life. If you find something of value in this podcast or this episode, please share it with someone who needs to hear it. Your support goes a long way in growing our community. [00:55:00] Once again, thank you for sharing your time and your energy with me and our guests. I'm your host, Adam Gamwell, and you're listening to This Anthro Life. We'll see you next time.
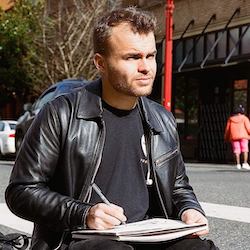
David Gray Widder
Doctoral Candidate, Carnegie Mellon
David Gray Widder (he/him) studies how people creating "Artificial Intelligence" systems think about the downstream harms their systems make possible. He is a Doctoral Student in the School of Computer Science at Carnegie Mellon University, and incoming Postdoctoral Fellow at the Digital Life Initiative at Cornell Tech. He has previously conducted research at Intel Labs, Microsoft Research, and NASA's Jet Propulsion Laboratory. He was born in Tillamook, Oregon and raised in Berlin and Singapore. He maintains a conceptual-realist artistic practice, advocates against police terror and pervasive surveillance, and enjoys distance running.
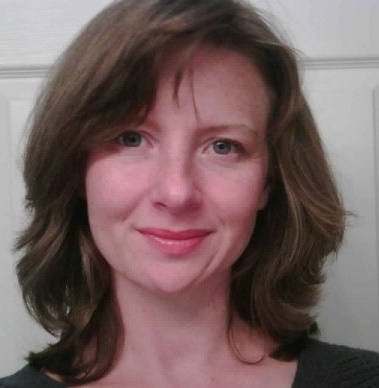
Dawn Nafus
Senior Research Scientist, Intel
Dawn Nafus is an anthropologist and senior research scientist at Intel Labs, where she leads research that enables Intel to make socially-informed decisions about its products. She is the editor of Quantified: Biosensing Technologies in Everyday Life (MIT Press, 2016), co-author of Self-Tracking (MIT Press 2016) and co-editor of Ethnography for a Data-Saturated World (Manchester University Press, 2018). Currently she is working on the fusion of energy and computing infrastructures, and low-carbon AI innovation.