How Tech can Democratize Scientific Knowledge with Eric Olson
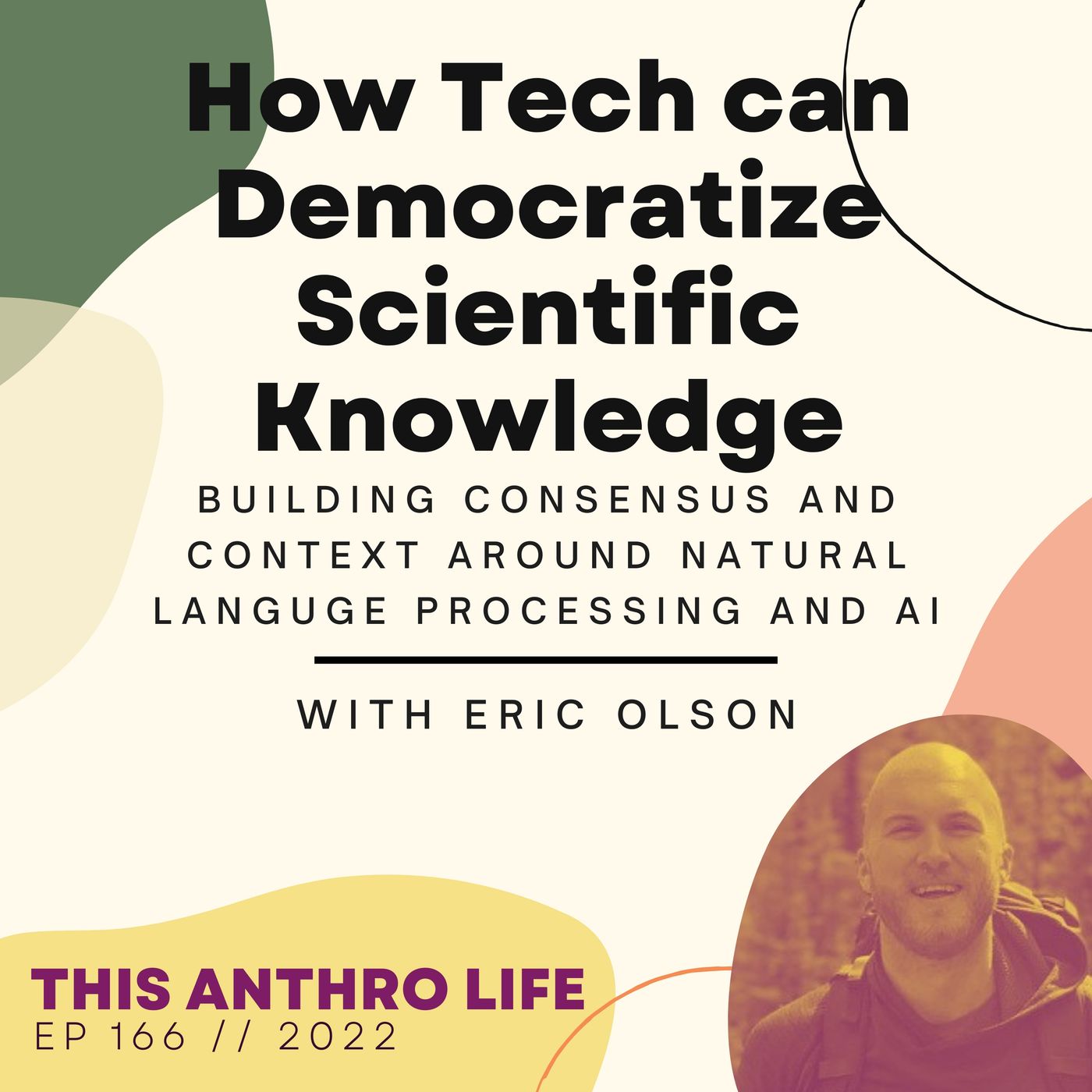
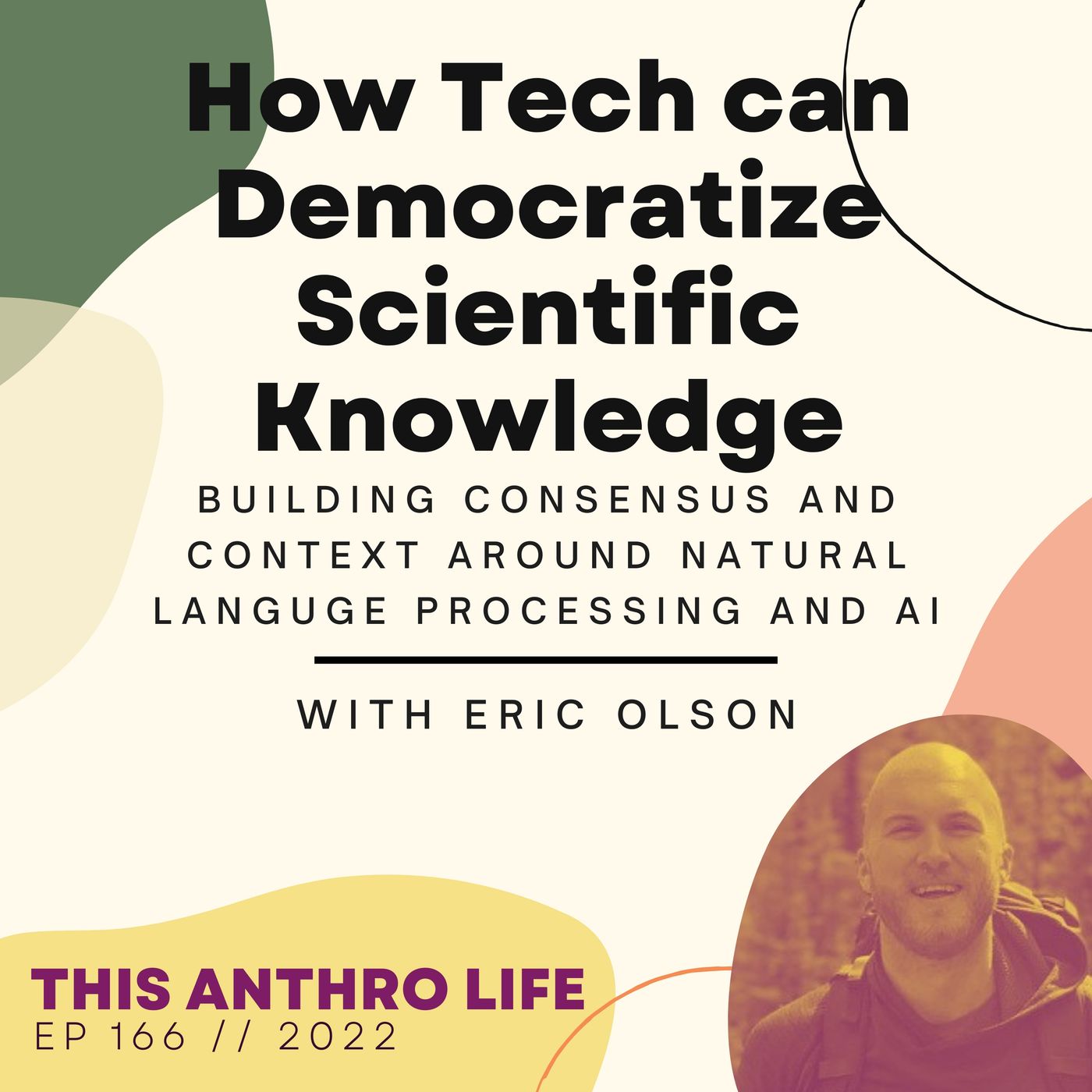
The vast majority of published scientific literature and new research is hidden behind paywalls. Worse, what few accessible papers available online are oftentimes written in jargon, i.e., specialist language that can alienate non-expert readers. natural language processing-based startups are working to change the conversation around access to scientific knowledge in impactful ways. One such startup is Consensus, an AI-powered search engine designed to provide users a view into what the research says with the click of a button.
The vast majority of published scientific literature and new research is hidden behind paywalls. Worse, what few accessible papers available online are oftentimes written in jargon, i.e., specialist language that can alienate non-expert readers.
Combined, these two issues make it difficult for researchers, scientists, and even entrepreneurs to build on new discoveries and for members of the public to access credible, peer-reviewed literature in the age of misinformation.
The good news is, natural language processing-based startups are working to change the conversation around access to scientific knowledge in impactful ways. One such startup is Consensus, an AI-powered search engine designed to provide users a view into what the research says with the click of a button.
In this episode, host Adam Gamwell is joined by Consensus CEO Eric Olson to talk about the company’s inception, the promise and new waves of natural language processing technology, and how Consensus is making scientific findings accessible and consumable for all.
Show Highlights:
[04:08] How Eric Olson got into natural language processing
[06:15] How tech can help users know what information to trust online
[08:10] The difference between giving good information and giving engaging information
[10:32] How Consensus attempts to disrupt the global search industry
[13:50] The current state of search
[15:32] How Consensus approaches partnerships
[17:07] On the size of Consensus’ corpus
[19:59] How natural language processing is evolving
[21:19] How Consensus fine-tunes its AI system
[24:53] On using AI generators to write papers
[26:47] How search platforms like Consensus can be built in a way that’s usable for laypeople
[30:32] Why context in AI is important
[33:05] The three things that differentiate Consensus from existing search engines
[39:37] What’s next for NLP-based technologies as a whole
[41:14] What’s next for Consensus
[43:10] On the hypothesis that AI can’t replace subjective, art-based roles
[46:12] Closing statements
Links and Resources:
- Check out Consensus
- Subscribe to This Anthro Life’s newsletter
- Connect with Adam via email
- Connect with Adam via the This Anthro Life website
[00:00:00] Adam Gamwell: Hello and welcome to This Anthro Life. I'm your host, Adam Gamwell. You know when you're watching the news or a YouTube video or a documentary and a talking head says, "There's scientific consensus that climate change is manmade," or "There is a preponderance of evidence in the literature that meditation is linked to increased feelings of wellbeing," have you ever wondered, well, cool, but I'd like to see that consensus or maybe be able to follow the trail of scientific claims made around mindfulness to be better informed? Well, I've got good news for you. Getting to the consensus behind the claims is what today's episode is all about.
[00:00:34] One of the big goals of This Anthro Life is to amplify the voices, tools, and technologies that bring our creative potential to life. And I'm really excited to be joined on the podcast today by Eric Olson. Now, Eric is the CEO of a startup called Consensus. Consensus is a search engine that uses AI or artificial intelligence to instantly extract, aggregate, and distill findings directly from scientific research. Sounds pretty cool, right? It's built around simple searches like "are COVID-19 vaccinations safe?" or "the benefits of mindfulness" or linking together ideas, such as “poverty reduction and direct cash payments.” It then searches across 200 million papers adding more every day and returns results that highlight the scientific claims made in each of these different articles. This is amazing for a few reasons. One: It doesn't tell you what to think. It simply shows you at a quick glance what are the majority of scientific claims saying about your query. And two: We're seeing the emergence of a new wave of natural language processing startups and tech that's aimed at democratizing scientific knowledge and access. What a time to be alive, right? Now, we're gonna get deeper into the why and how of Consensus in our conversation today.
[00:01:47] So to kick things off, I just wanna lay out two big challenges that tech and services like Consensus face for us as listeners to think about, whether we're social scientists in academia, researchers or designers in industry, or just a good old-fashioned public citizen concerned with literacy and public debate. With the rise of research and information technology, it's surprising when you stop to think about it that we haven't also found ways to build consensus around expertise. And there are a few challenges here at play. One is that peer-reviewed scientific literature in journals like Nature or Cell, if you're in the biological sciences, or American Ethnologist, if you're an anthropologist, are behind memberships and paywalls. So if you're a student at a university, you may never notice this since your department or university often pays for that subscription. But depending on where you go to school, you may have noticed that you only have access to some journals but not others. So this is problem one. Scientific literature is locked behind a paywall that most individuals and even some organizations can't afford. Then the second is an issue around accessibility. Peer-reviewed literature is often written in heavy jargon, that is, specialist language that makes it difficult and time-consuming for non-expert audiences or readers to digest. Now, this is more of a stylistic challenge whereby academic writers follow along with and reinforce communication styles that work for the academic system but not for public access. Now, sidebar: It is debatable, of course, how well this kind of jargon works for academic systems, too. And this is actually one of the reasons that I started This Anthro Life back in 2019. Whoa. Nine years ago at the time of recording this episode in late 2022. Okay. So these two issues contribute in a big way to why we haven't seemed to be able to find ways to build consensus around expertise despite having mountains upon mountains of scientific data and research in our collective human database.
[00:03:42] Now, Consensus is working to change this conversation in really fascinating and impactful ways. And as we'll discuss in today's episode, we also need to ask ourselves and ask of society what we want and need out of access to scientific knowledge. So we'll be right back after a quick message from this episode's sponsor and can't wait to dive in with Eric Olson.
[00:04:08] You know, to kind of kick us off, I'd love to get a bit of a sense, we kind of think about your superhero origin story, you know, the bit of your tale of like how you kind of came into the arena that you are now around the idea of using, you know, natural language processing and aiming to disrupt the search engine industry, which I love this idea. But tell me how'd you get into this space in the first place.
[00:04:26] Eric Olson: Yeah, thanks for having me, Adam. Great question. So the real origin of it is, you know, I come from a family of academics and scientists but I am not one myself. And it's kind of created this outsider complex within myself that made me really, really interested in science but made me an incredible amateur at consuming it. So I found real value in consuming content that had scientists who are breaking down what the research said about a subject in a way that a non-academic like myself could understand. So it was from getting that value that like, it was actually like six or seven years ago, came up with the idea of what if there's a way to automate this process and what if there's a way to get answers to these types of questions that I have on demand from research, from peer-reviewed sources.
[00:05:12] And then fast forward five or six years and it was actually my co-founder, Christian Salem, who I pitched the idea to all those years ago. They came back in the middle of COVID and was like, "You remember that idea you had? I think the world really needs this right now." And that's kind of how we got started on our journey. And then the real light bulb moment for us was after that, we started to dig into what was the state of the technology. Could technology actually solve this problem? And then learning that natural language processing, in the advent of these large language models, had really just occurred. And it was really this kind of perfect "Why now?" synergy of societal demand and technological feasibility.
[00:05:51] Adam Gamwell: Interesting. And it seems like on one level, too, some of the best science and scientific discoveries are some kind of synchronicity, right, where it's like the tech came together with the moment at the time and what people were looking for. So it seems like it's a good triangulate there, you know?
[00:06:03] Eric Olson: It's lucky in a lot of ways, right? Like things are more random than we wish them to be. And both of those things had, me and my co-founder, had nothing to do with us, right? They were completely things out of our control that kind of coalesced to make this possible.
[00:06:15] Adam Gamwell: You know, I mean, it's also like this idea that ethnographers talk about kind of from human sciences, anthropology, sociology, that some of the best power and innovation work comes from when we can see those connections that other people might miss. And so, I'm interested in thinking about this idea and in terms of these pieces that came together. I mean, that's I think one of the powerful elements here, too, is that as we have this kind of perfect storm, as it were, for this need, I mean, one of the pieces that stands out, you're talking about, you know, six, seven years ago up to today, one of the big topics that comes to mind is things like the rise of misinformation online as a challenge point in terms of, you know, fake news is the meme or hashtag of the day, right? But just this idea, I mean, how did things like this filter into that kind of thinking process? And I think that this is, I think one of the big challenges that a lot of people face today is I don't know what information to trust online. And, you know, how can technology, how can tools help us do that, especially when sometimes those seemingly same tools are the ones that like, make it so we can't trust it in the first place, right? Like algorithms and advertisements. So how do we think about this kind of model? How can we like, set the stage of what is this problem space that we're coming into that we wanna solve for?
[00:07:26] Eric Olson: Yeah, no, no, really well said across the board. So I think it might be helpful to like first just like level set on exactly what it is we're doing and then I can kind of use that to segue into answering your question a bit. So, yeah, we are Consensus and we're a new search engine that allows you to type in plain English questions and be returned relevant findings from peer-reviewed literature. So basically, the idea is instead of going to Google when you have the question of "does magnesium actually help me sleep," you can type in that exact question. You don't have to do any special, you know, Boolean searching or anything. You can actually just type in that question and our NLP will look through research papers and try to find claims being made about that question and deliver them to you in this nice, aggregated, easy-to-consume list.
[00:08:10] And yeah, to go back to your question, yeah. This is another part of our kind of "Why now?" story. Obviously, the societal demand, and the clear currents of misinformation, and that people were frustrated with their inability to get good information. But it was really, you know, a key part of this is kind of like identifying why that is the case. And you said it really well yourself that it's like, you know, we want technology to solve all these problems, but as it turned out, that technology was what was creating a lot of these problems. But there's one real key component of it, too, that is driving that is that, you know, most of these applications that we use and websites we visit and specifically, you know, if we're talking about Google, the way that we search for and consume information, all this information is passed through this advertising filter. And that means, you know, explicitly sometimes just being shown ads if you typed in "does magnesium help me sleep?" You'll get a bunch of ads for magnesium supplements. But the undercurrent of it is that because their incentives are to sell more advertising space, what they optimize for is continued engagement. So, you know, Google or Facebook or any of these places misinforming you isn't some giant backchannel conspiracy theory of people in rooms and suits being like, "We want to try to make Adam believe something that isn't true." It's really just these organizations, you know, following their incentives and optimizing for what's gonna make them the most money. And that's continued engagement 'cause that means continuing selling ads to people's eyeballs.
[00:09:33] So in turn, that creates systems that are not designed to give us good information. They're designed to give us engaging information. And the problem is that when algorithms try to learn what's engaging, a lot of times what they find out that what's engaging is content that is, you know, flashy and fiery and controversial. So in turn, you get shown lots and lots of that that has nothing to do with how factual the information is. So our real pushback on that is that we think that the future of search has to be in this premium, verticalized subscription model 'cause that means that, you know, we're not selling advertisements. All of our incentives will just be to deliver you the best product and deliver you the best information possible. And the hope is that with NLP taking off so much, that products can get so good at these services that people will be willing to pay a small subscription fee for them. And that's when you could actually see this inflection point of some pushback on these free, advertising-based tools.
[00:10:32] Adam Gamwell: I mean, that's super interesting and has a bit of that, if I may, David and Goliath theme to it, right, where —
[00:10:38] Eric Olson: Certainly.
[00:10:40] Adam Gamwell: How does that feel? I mean, I think both an exciting but a challenge point, you know? How do you kind of approach this idea of like, I think it's, what you're saying is super resonant, that there is this ongoing challenge point that the way we're presented data is not based on what's most factually correct or that even necessarily answers my question, but it's based on an advertising model that has made a multibillion-dollar global search industry. How do we even disrupt that, you know? And so, I think it's this really interesting question. I mean, maybe you have like, to bring a metaphor, you have this small stone maybe of NLP to help us.
[00:11:10] Eric Olson: I mean, that's exactly what my answer is gonna be. Like I'm not here to say that we're, you know, on the precipice of disrupting Google and making them like, you know, really taking away all this, all the money that they're making. But the way to make a giant change is, you know, to kind of follow that David and Goliath example somehow and find your wedge, find your stone. What is the thing that you can do really well and start small there. And for us, we think a perfect place to start is with scientific literature 'cause it's, you know, the information that's the most valuable source of data in the world. And there's insights to question, all sorts of questions that people have and there are insights that are really hard to get by using the currently available tools. So we think it's a great place to start to maybe, you know, disrupt the tiniest bit and then go from there, right? You can't start somewhere. If you just try to show up and say, "Hey. We're gonna knock down Google," you have no shot to do it. You start somewhere, you get really good at that, you build an awesome product in that space, and then we'll see where it goes.
[00:12:03] Adam Gamwell: That makes good sense. And I think also wise, right? It's like we do what we can but like picking that right movement. So I think it's, that's really compelling that scientific literature kind of functions as that fulcrum, right? That's the piece we're using to then be able to rethink how search works, you know? And like, so it's a very specific example. And so, to kind of set the stage here in terms of the problem here, I think there's something interesting, right, that. So I've gone through grad school, a lot of my colleagues have. And, you know, one of the big pain points, like you don't notice it when you're in school is that, you know, all the journals that you're reading for research are behind paywalls because usually your school pays for those. But it's interesting like as I spent more time talking with other colleagues across the years in different disciplines or they went to different schools that had less funding, they wouldn't have access to some of the same journals I found. And that was interesting to note. It's this privilege of information. And that feels worse when you think about it as scientific information, right, as peer-reviewed literature that we don't have access to.
[00:12:55] So I wanna think about that with you. Like this, the impact of like inaccessible or making accessible scientific information. And I mean, I, you know, will just straight up say I think the current model is broken, right? Like behind a paywall where a lot of research sits. I mean, this is also interesting in conversation with the fact that at the time of us recording, the Biden administration just passed this law that at the end of 2025 into early 2026, any federally funded scientific research has to be immediately available upon publication, which is really interesting. And like saying that feels kind of crazy because it's 2022 and we're still a few years away from that even being a thing, right? So that's, it's crazy to realize up until this point, scientific research is still behind a paywall for the most part, especially the most prestigious things like Nature or Cell or, you know, science or biology journals. Anyway, so I'm rambling now, but tell me about this process and like how we can think about this idea in terms of why we need accessible information and like, and how do we think about this, the current paradigm, today.
[00:13:50] Eric Olson: Yeah. You know, and when we've talked about what our problem statement is, the broad in a way that we get, you know, people's eyeballs to light up and what like the broad vision of it is this, that search is broken. It's really hard to find good information. Like that's the way to paint it with this giant brush. But what we call, like our sub-problem statement has been that, you know, the most valuable and insight-filled source of data on the planet is sitting behind paywalls. But now that they're just sitting behind paywalls, because of that, it's only consumed by the same people who create it. And there's no way for somebody like myself to engage with literature unless I'm a part of a university and I'm doing research myself. And that's a shame on a million different ways. One of them being like the very obvious just like economically, the way the system is just broken because it's, many of these things are funded by public dollars yet they're not accessible to the public. And that just is incredibly backwards.
[00:14:41] But the good news is, the trend is in everyone's favor. Like you said, that the Biden administration just passed this legislature. And even before that, the trend has been moving toward making things more open science or making things more open access. And our data partner, they're called Semantics Scholar, and they're run out of the Allen Institute for Artificial Intelligence in Seattle. It's actually Paul Allen, the Microsoft founder's AI research arm. And they're basically a Google Scholar competitor. They're a research data aggregator. And they try to focus a lot on open access papers. So a lot of our corpus actually is entirely open access. Not all of it is. We can still do our analysis over the papers. But if you're to try to go to the full text from some of our search results, you will run into the paywall unfortunately sometimes. But a good chunk of our papers are open access. And like you said, the trend is gonna continue to be working in our favor.
[00:15:32] Adam Gamwell: Very cool. Thinking about that actually, the idea in terms of, I was curious to think about this, and it may be through Semantic Scholar, but how do you approach partnerships in terms of like working with, in terms of how does like Consensus plug into partnerships in this regard in terms of aggregators versus search engine versus kind of the NLP, the tech stack that helps put these pieces together? And with that, I'm curious, you know, so as an anthropologist, do we get anthropology journals as part of this as well? I know they're not as fancy as oftentimes biology or chemistry journals. They don't get as much press. But I'm just curious in terms of that, like how do you select for the kinds of articles, journals that are included in the kind of the search process?
[00:16:09] Eric Olson: Yeah. So to kind of start with like the partnerships question, we're incredibly fortunate that we're operating in a space with lots of research organizations that are nonprofits and that are, can be fairly open to partnerships like this. There is like a big caveat though that you, many times you need to basically prove that we have mission alignment and stay true to those ideals of promoting open science and promoting access to scientific literature. That is basically how we got the partnership with Semantic Scholar was, you know, they're willing to do partnerships with other research organizations basically without any vetting. But we had to go through this vetting process as a commercial entity to say, "Hey. Here's how we're gonna be using it. Here's how you benefit. And here's how we push forward your mission." We've really tried to work hard to abide by a lot of those values so we can be attractive to have partner, I mean, one, because we also want to abide by those values, but also it has the other benefit of being able to partner with a lot of amazing organizations that have incredible mission statements of promoting access to scientific literature.
[00:17:07] As far as anthropology journals, I don't know that answer off the top of my head. We have pretty darn good coverage. We have about 200 million papers in our corpus, so I would be shocked if there are not some anthropology journals as a part of it. Unfortunately, I don't have that answer off the top of my head. And then as far as like selecting, it's really whatever we can get our hands on. We're not trying to select by domain. The way that we've trained our algorithms is to try to be domain-agnostic of extracting findings from papers. And I think one of the great surprises of our early product is how well it does with nonmedical, healthcare-related questions. I publish, if you sign up for our product and become a free user, I send out a newsletter every week that's like interesting thing I learned this week, query of the week basically. So it's like, it's a question that I asked and what the results were. And this week's was, "Does the death penalty reduce crime?" And our product did an awesome job of surfacing research findings about that. So I'm using that as an example that the domains can be a bit, you know, less hard science than you typically think of when you hear peer-reviewed literature. And our product can still do a pretty decent job of finding relevant conclusions about those questions.
[00:18:11] Adam Gamwell: That's super interesting. I think something that stands out to me here, too, is that's really awesome to hear that the algorithm that you put together is able like kind of thinking like, a thinking domain agnostically I think is really important. It's making me think of like, you know, I dunno, my dreams of being a Zettelkasten journaler that I can like connect ideas together with double brackets and like be whatever subject agnostic and just connect ideas as they link together. And also, I mean, more like how the human brain works, too. So I think that's something else that I think gets me really excited about the possibilities that, you know, kind of what the emerging world of natural language processing can do is that we can train it and train ways that'll kind of use the same pathways that our brains might, right? That we don't get stuck in silos of like, I only want to get journals that are from biology or just from anthropology or whatever it is. But actually the death penalty question is interesting in terms of probably the diversity of results that you got back.
[00:19:03] I'm also thinking of another example. I saw the blog you recently did on the use of psilocybin medication like based on Michael Pollan's How to Change Your Mind series. And like that was a really cool, I think, example, too, in terms of showing the, for lack of a better term, the pop culture application of asking of scientific literature. I mean, that's really what Michael Pollan's work is all about, right? And death penalty is a great question, too, in that regard. 'Cause also the other thing, too, is even that space, how do we even know how much is out there, right? And so, thinking of the power of that we can use big data in ways that the human brain doesn't quite do so well. Or just like literally searching at LexisNexis, right, or bioRxiv, you're only gonna see so much in terms of returned results that you can read. But again, this idea in terms of how can we kind of cross those boundaries, I think is really exciting. So is that what you found, too, that, is it surprising how good natural language processing has become? You've kind of been steeped in it for a while, but, you know, how are we seeing it evolve also, you know? Even through Consensus, right, as you think about partnerships and what you're searching for, how have we seen that change?
[00:19:59] Eric Olson: Yeah. Even though we work in the world of NLP, it continues to pleasantly surprise me every day, I would earnestly say. What you said is really interesting of like how it kind of operates in some ways the way that the human brain works and the real advent of the new technologies, they're called large language models, and the real unique feature about them is that they come pre-trained. And they come with this underlying knowledge of how human language works. So the big one that you've likely seen in the news and listeners have probably seen on Twitter or something is GPT-3, OpenAI's model. It is part of the underlying technology of DALL-E, which is that you type in a prompt and you get back like an art image. People have been loving that on Twitter. And that is trained on basically like the entire internet of articles, of text articles. And the way they teach it to understand language is they showed examples of articles with certain words blanked out, and they try to make it predict what word goes in those spaces. And then you show that literally billions of examples and over time, they can learn how to accurately predict what word should go here. So it actually understands like the whole context of what's going on around it. And yeah, it's shown the entire text before it makes that prediction. So it's trying to understand text holistically in the way that language works.
[00:21:19] And then what happens is when you try to do one of these specific tasks like we're doing at Consensus, you do a process what's called fine-tuning, and you give it more custom training data specifically for the task that you're trying to do, and then you teach it how to do that task. But it comes with that underlying knowledge first. And if you think about the way a human would learn how to do a task, it's very similar to that, right? If you're being taught something, you don't come in as this just blank slate. You have all these patterns that you've learned about other tasks you've completed in your life when you come to a task to learn how to do it. So it really is in some ways mimicking the way a human would learn how to do something. And in the context of how we do it, basically, yeah, there's a pre-trained model and then we give it examples of, we hire scientists to go through research papers and mark up what are authors making their claims. So basically, it's just a bunch of ones and zeros next to sentences where it says zero - this is background information, zero - this is methods, zero - this is more background information, one - when they say these results suggest that, so on and so forth. And then when you give it enough examples of that, you can feed that to a model to learn how to pick out those sentences.
[00:22:27] Adam Gamwell: Very cool. Even this idea, I think is really exciting in importance of context, right? And part of it is like, hey, how do we train models but then also recognizing that? I love the way you said that, too, where it's that we're never just born as blank slates, right? We always have a set of pattern recognition and context that like shape who we are and like the fact that we are able to build technology that follows that, too, is exciting. You know, obviously it means we gotta watch it.
[00:22:50] Eric Olson: And it makes it super flexible, right? Like it makes it so you can teach it to do a whole host of tasks.
[00:22:55] Adam Gamwell: Yeah. I think that's right on, too. And I think like that's in some of the most important power. It's like you, at the same time as we're through neuroscience, through psychology, really coming to understand the plasticity of the human mind, right, and the fact that we can't even reshape neural pathways through training, through different kinds of lifestyle even. That we can see this kind of happen also on a technological software level is really fascinating that they're kind of happening simultaneously, which is interesting. And I know that's because we just learned to see one and then we can see the other one now or kind of how that happened. Maybe chicken and egg kind of problem there. Kind of funny to see some co-evolution there, I suppose. So I'd love to kind of think with this idea, so as we're training our software and also working with scientists, it's a very cool point, too. So we've got humans as part of that.
[00:23:34] I mean, it's one of those things that I've conversation I had with Byron Reese on the podcast a few years ago. And more recently, he's the CEO of Gigaom and like did a podcast on voices and AI and was really, really into the role of AI in changing how technology works and like obviously going into things like NLP. And, you know, it's this interesting idea that a lot of times that there's a common kind of, you know, pop cultural notion that AI is something that will become generalizable, that it will become essentially conscious at some point, right? And like this, you know, filters into our, you know, bigger fear narratives of like the Terminator and the Matrix and things like that. But realistically, you know, most models say AI can't do that, and it won't. And even the way that we're talking about this in that AI often at this point needs to work in partnership with people still, right? Like it's learning how to interpret context with humans because we're the ones that do it without even thinking about it, right? I think this is like an important piece for folks to like pause and think on, is that AI is super powerful and it like gives our brains a superpower to connect ideas. But really it's also, to your point, is based on a lot of how we do interpretation as people and learns from that. So it is interesting, too, that it's that as much as we're students of the way we can connect knowledge, AI is a student of us also.
[00:24:43] We're gonna take a quick break. Just wanted to let you know that we're running ads to support the show now. We'll be right back.
[00:24:53] Eric Olson: Yeah. I was on a podcast the other week. We were talking about NLP and he was talking about it as like, you know, he was reading an article that they're gonna have to work to like fight plagiarism in schools because students could use AI generators to write papers, which totally can happen, right? And AI can do that now. But I was saying, my response to them was, if you're a student that's trying to do that, you know, there is like a prerequisite step in some ways that you have to get a bunch of training data of A papers, show a model to then learn how to do an A paper. So the work that it would be required to, you know, fine-tune a model to do exactly what you needed it to do for your class, you know, is way more work than just writing the paper yourself. Now, with that said, like a generalizable generation model could write you like a paper that could pass as a human paper, but it probably isn't very good for your class. And in order to really get it specifically at the task in the way that you would need it written for your class, you would probably need examples of that. So a prerequisite for having a model spit you out A papers is to write the A papers yourself.
[00:25:55] Adam Gamwell: That's a good point, right? So like you can plagiarize. But.
[00:25:59] Eric Olson: Right. Exactly.
[00:26:01] Adam Gamwell: Yeah. It's like hiring someone else to do it exactly, right? But that's, I think that's really interesting. And I think that's right on where there can be a fear of adopting technology, especially one like that feels like it gets close to home, right. Like how am I writing, you know? But it's based kind of in the similar concern that we kind of talked about up top where there's a lot of mistrust online on one level. Like I don't know if I can trust the information. And so, we've also seen, you know, in the past 10 years, the rise of plagiarism checkers like in Word. Microsoft Word has one, you know? Google will look for it, too. And so, I've even seen AI writers that then also will plagiarize check itself, which is interesting because it doesn't know if it's plagiarizing until it looks and says, "Okay. I wrote this thing. Oops." "Yep. I borrowed that from Wikipedia." Which I think is interesting as well. So it like, it doesn't know it's plagiarizing until it writes, then it checks, you know, oftentimes, or the ones that I've seen anyway.
[00:26:47] But I think that's a really interesting question, too, in terms of, there's the work that we have to do in terms of providing more access to scientific literature, making it more accessible and easier for folks to get, especially for laypeople, right? Like one, we already looked into this question of the paywall problem, but then how do we make this usable for your average layperson, too, who's never gonna get a master's or PhD. Doesn't need one, doesn't want one. But they're interested in like, yeah, is our COVID-19 vaccines effective, right? What are the benefits of mindfulness? These are some examples on your website. I thought they were really good thought starters. So how do we think about that? Like how do we help build this in a way that's useful for everybody?
[00:27:25] Eric Olson: Yeah, it's a great question. And I think that it's finding the balance of not oversimplifying and perverting meaning while trying to use these tools to make things consumable and digestible and easy to use. I think a real big emphasis on that last one of easy to use 'cause that says nothing of the digestibility always of the text that you're showing. So we'll kind of tie this all together that what we're doing is we're not generating any text 'cause we think that is a fairly dangerous road to go down of trying to summarize what a scientist is saying without any checks on what does the source text actually say without being able to show that to the user. So we want to do our best to always just extract the information and show them a real quote from the literature.
[00:28:12] So where I'm going with all this is that is how you use the tool to make it easy. You try to find the sentence where they're answering the question and then just extract it. And you might be sacrificing a little bit of digestibility 'cause, you know, scientists still love to write in jargon and that sentence may be jargon-filled. But we kind of think that, you know, this is by no means perfect and we're gonna continue to iterate on it. But we found that to be this kind of nice balance of using these tools to make things easier by finding the answers but not going overboard and not oversaturating and oversimplifying it by doing all this like super advanced generation where we could be totally perverting the underlying meaning of what the scientist is trying to say. So I think like, in total, my answer is like trying to find that balance of where can you use these tools to circumnavigate things and make things easier while not going overboard and oversaturated and oversimplifying.
[00:29:03] Adam Gamwell: Yeah, that's fundamentally important, too. And even the software itself, I actually really appreciated this, it has a beta tag on the website and it says, "Do your due diligence from this work." You know, it's like, don't just take this as gospel but, you know, still be a scientist, still be scientific in your thinking and say, look at this, and then continue to look around as part of your process. And I think that's actually really fascinating, too, because I think you're a hundred percent right where it's like in the world of UX and usability, it's like we wanna make things easy to use for users. We want them to be frictionless and be a good experience. But, you know, especially in this case, like when we're looking at also scientific data, I think it's really, really fascinating and important that the point that you've noted that it's, we have to make sure that we're not either oversimplifying the complexity of an answer but then also that means we're doing interpretation of it, right? And that then may change, but we are then changing the meaning of, if we change the wording to make it sound more or less jargony, we realize we're adding a second layer there in terms of interpreting what we're seeing. And that's an interesting and like big conundrum that, you know, whole different like can of worms as it were. But interesting to think about.
[00:30:01] Eric Olson: Yeah, we're actually training some dejargonizing models. But we were just having this conversation earlier today about how we want it, you know, we're still a ways away from having that in the product. But our idea for how we'd want that in the product is a little button that lets you toggle that on. So you are, you're basically saying, I am acknowledging that now I wanna see this slightly more simplified version of these claims. And, you know, you're very acutely aware that you should be doing more due diligence when you're interpreting one of those results with the little like toggle bar at the top right of the screen.
[00:30:32] And yeah, appreciate you calling out the beta tag 'cause, yeah, we think that's important. We're still early, we by no means do everything perfectly. And even if we did extract all these answers perfectly, like it still is important if you're gonna be, you know, making an action item in your life because of one of these answers, you should probably pop open the paper and read all about it. Like it's great to be able to get a landscape of evidence really quickly. That's why we built the product. But context is always incredibly important. You should do your due diligence when you're going through these findings. And then, yeah, to your point about frictionless, yeah, like that is we've tried to make the product really easy to use 'cause that's a way to make it approachable without having any risk of anything to do with perverting the science, right? Like just in the login flow and the way you can search and the try searching buttons and all of those things like, right, those are things that are completely outside of the information we're showing. They're usability in a much more like aesthetic way. So we're trying to do everything we can to be really good at those things 'cause those are ways we can make this more approachable for anybody without having to worry if we're dumbing down the science too much.
[00:31:35] Adam Gamwell: That's a great point. And I think super valuable, too, because, you know, one thing I often hear, too, is that, you know, working in consumer research, whether it's working and doing kind of design research with different clients, that there can be this, I don't know, fear is too strong of a word, but just, you know, if folks are not used to scientific literature research, like it's a scary space, right? Because it is. It's jargony, it's behind this weird paywall, it's complex. You know, how do I understand it? And so, I think there's, you know, to your point, like a real value in making the experience, the digital experience of coming even to the search itself welcoming.
[00:32:05] Eric Olson: Google Scholar hasn't changed in 20 years. It's the same interface for the past decade.
[00:32:08] Adam Gamwell: That's so true. That's so true. And it's hell to try to search something on there, right? You can't get like internal parts of papers. You can get titles, but there's nothing in terms of really finding a way in. So to me, also, this really fills a big gap like that, right, in terms of broadening that question of access. But then also the rise also in, you know, natural language itself. I mean, this is something that I, as a consumer side, came into being interested in this like with calendars, right? Meet with Eric on Tuesday at 2:00 PM, you know, like I could type that in then it would add an event to my calendar. I thought it was super cool, you know? I think then being able to do this in asking scientific questions is incredible, right? What a really interesting way of having to then think, okay, you know, how do I formulate my hypothesis in a three-part question, you know, for a paper? Way harder than just saying, yeah, what are the benefits of mindfulness? And then kind of returning results in that space, I think is really interesting and important because that also play, I think, an important role in accessibility. You know, where folks feel like they have a point of access they may have not had otherwise.
[00:33:05] Eric Olson: Yeah, we think there's three main differentiators between us and a search engine like Google Scholar. And we think it's like the three things that are the worst part of that experience of using Google Scholars. So one is exactly like you said of the actual trying to get information related to your question. Google Scholar does not allow you to type in a plain English question. As you type in your research question, you're not gonna likely get back very relevant results. You have to do this like, you know, kind of insider exclusive Boolean searching to get it to come up with what you want. And then there's that next part of all they do is just give you links. Which, you know, if you're doing a giant lit review and you're a PhD and, you know you have to go dedicate, you know, 48 hours or 50 hours of your next week to do that. Fine. Like whatever. But, you know, you’re an everyday consumer who just wants to ask a question and get it from good sources, you're not about to go through all those papers and spend considerable time. So we wanted to make a way that makes it easier to get information from those papers where we're not just delivering UX, we're actually surfacing the insights to your question. Now, if your then objective is to go deeper and this is a better way to surface papers to look into, great. But if it's also just a way to quickly get an understanding of what the research says about a subject or a question, also great, right? And then the last one is, yeah, a little more subjective, but just their interface sucks. And we're gonna always continue to try to build it to be a real product experience and not just some clunky academic search engine.
[00:34:29] Adam Gamwell: It matters, though. I mean, like Apple really kicked up like consumer design language as needing to be important, you know, with iPhone. And so, I mean, through today it's that like, it's interesting to see there's been such a broad respect now for things like color palette and typography. And this matter, right? And like where is the affordances that I can easily see, I can click on on a website. Make a difference, you know? And like, again, having these come together around scientific research, I think is like, that itself is also somewhat revolutionary, I guess is what I'm trying to get at. Like that we don't often have accessibility front and center as a point of value, as a mission orientation for scientific research, which I think is super important in that regard.
[00:35:04] I think that another thing stands out that I'd love to hear about is, you know, we talked a bit about Semantic Scholar. But then you've also talked about, I've seen your website, like other partnerships like with SciScore. And this is important because it's how do we build trust in what we're finding. And so, this partnership, tell me a bit about this, too, or, or if there's other ones like this that are kind of help put together the bigger web of how do we build the tools and the trust.
[00:35:25] Eric Olson: No, it's a great question. Yeah. We're extremely honored and privileged to be able to partner with SciScore. So they are actually another private company, but similar, we have a similar mission statement and are after the same things. And what they've actually done is it's the largest analysis of scientific methods ever conducted. And they did an analysis, I believe it was 1.5 million papers, and looked at the actual methods that these studies undertook, what percentage of them were randomized, blinded, had ample statistical power, had diverse population sets. And they basically graded the papers, and then used that to grade the journals that they came from. So the whole thing is just a giant pushback on traditional bibliographic ranking systems. The one everyone knows about is impact factor, right, which just is a measure of influence and citation counts of journals. And this is basically a pushback on that. And they actually publish their own paper that showed that it was completely uncorrelated with impact factor, which is a pretty big, yeah, red flag for those who are using impact factor. And it makes sense, right, 'cause it's actually looking at what the researchers within these journals were doing.
[00:36:33] It still is a proxy metric. Like just because something comes from a journal that typically has really high standards doesn't mean that a given paper doesn't. And that is a slight limitation of those tags that we have in the product, that it getting a good score just means it's from a good journal. It doesn't inherently mean that that paper is better. It makes it much more likely that it is, but doesn't inherently mean it. But it is a real step forward in terms of these proxy metrics. And then the future is being able to take some proxy metrics, also looking at the study itself and looking like what were the actual methods of this paper, kind of rolling that up into one and saying, you know, what is the rigor criteria of this score? What is the reproducibility of those findings? That's something we are actively working on and really hope we can get into the product.
[00:37:17] Adam Gamwell: That sounds amazing and super interesting and important, you know, especially 'cause I'm trying to think that, my partner is a microbiologist and so that's how I learned about bioRxiv, you know. But this is an important point in terms of like the reproducibility and that as like a product roadmap feature, I think is super interesting, too, in terms of how could we like, ask these broader questions of methodology, which is something else, again, not really done. So I appreciate how, up top, we were talking about this idea that we're working with scientists with some zeros and some ones to kind of mark where we're talking about claims. And then on, this is like kind of another second, but just another step in terms of bringing in the questions of methodology, how those are discussed, what do they look like, and how do we rank that. And then also what might that mean for things like reproducibility of an experiment. Super interesting.
[00:38:01] Eric Olson: And can we automate that.
[00:38:02] Adam Gamwell: Yeah. Can we automate that? Like sign me up. That sounds really cool. And maybe we can use that to then do a little debunking of some of the behavioral science that like —
[00:38:10] Eric Olson: Exactly. We've actually met with the group, the Center for Open Science, and they've done some awesome work in this world. And the head of the Center for Open Science is Brian Nosek. And he is actually most famous for, one of the things he's most famous for, he is an amazing, amazing figure in this space, but one of the things he's most famous for is debunking the psychology study about power poses and how if you stand with like your arms and legs really wide, you're more likely to succeed in an interview. If you stand like that beforehand and then go in, you're more likely to speak well or speak confidently, do well in interview, public speaking. And he showed that that was not reproducible. And then that was a kind of bogus finding. He's been a huge pioneer in debunking some of these behavioral psychology studies. And we've talked with him about these reproducibility metrics and how we could actually incorporate it. Another example, we're not as formal of a partnership where we're using the data like we are with SciScore in the product, but it's a team that we meet with nearly monthly and continue to talk about these things and are privileged to be associated with them.
[00:39:13] Adam Gamwell: Very cool. This is just like a giant nerd fest of awesome that I know all the listeners right now are being like, man, how do I get in on this? This is great, to be able to plug into these questions.
[00:39:23] Eric Olson: We are totally free for now. It is totally free to sign up and create an account. So head over to consensus.app and create an account and ask some research questions. And yeah, a giant nerd fest of awesome, we might have to put that as like a tagline in the product.
[00:39:37] Adam Gamwell: You heard it here, folks. Giant nerd fest of awesome. No, totally. I think, and that's great actually, I just signed up myself, too, so I am excited to be digging around and see what we can find here. So I think kind of as a, "What's next?" question. I mean, you're kind of pointing a little bit or hinting a bit about what might be down the product roadmap. But, you know, this bigger question, too, of like, we're around the precipice of this wave of, you know, NLP-based technologies and startups. And so, where are we going? Like this is a really interesting space, right, where I think we're in the next frontier in terms of what tech can help us do around NLP. And so, what are you most excited for in this space? Like whether it's with Consensus or just what else you're seeing that's kinda shaping the industry or what we could do in the next five, 10 years.
[00:40:17] Eric Olson: You know, I'll give you an answer that applies a bit to Consensus as well as like, as a more broad answer of like what is to come. And apologies that this isn't totally revolutionary and is actually what we've somewhat talked about before. But what I'm probably most excited for is how these will be used to build really, really amazing specialized search tools because of the ability that, you know. I think they're gonna start to pop up in certain verticals. You know, I'm biased 'cause we're popping up in the science vertical. But there'll be other ones in other verticals that will build, use these tools to build amazing information retrieval, information synthesis engines where you can type in questions or do all these different types of searches and instantly be returned interesting results. And I am just so excited to see the innovation with products like that 'cause I think that is our opportunity to really change the landscape of how we take in information. If these products can actually become good enough that they can operate on subscription models, they have a chance to change the way that the world consumes information.
[00:41:14] And as far as, yeah, like what's to come with Consensus? You know, I've talked about some of this, that reproducibility score is something we're actively working on. And another thing we're really excited about is trying to do like, I kind of mentioned that like toggle button of potentially dejargonizing is trying to figure out the right ways to introduce some synthesis into art results. So like right now we're pulling out the quotations and we want that feature to always be available to people 'cause it's really valuable. It's nice and raw. We're not perverting any meanings. But we do want to experiment with ways to synthesize some of these results and give people maybe even quicker snapshot of the landscape of evidence just on one summary screen. Lot of questions to answer there, and there's gonna be a whole other things to consider when we do that of making sure we're not perverting meetings and representing things that shouldn't be represented. But there's definitely gonna be a place for that. And these NLP products are only getting better at large scale synthesis across a bunch of different sets of results.
[00:42:11] Adam Gamwell: Yeah, that makes me super excited, too. It makes me think, too, 'cause there's been a, you know, like explosion of read this book in five minutes, summary book services, you know, and oftentimes those are then written by people, right? They're like human-made syntheses.
[00:42:24] Eric Olson: And that's where it all started, right? Is like we saw like whatever products you've seen that are like manually curated products around like text summarization, those are gonna become NLP companies in the future. So like Blinkist is a perfect example, right? It's the book summaries. Those are manually curated right now. There's no world where in some order of time that isn't gonna be just done by machines. And like those are the types of products that we're gonna see pop up. A really interesting thing that I've thought about before is like the place that we're seeing a lot of these models take off early right now and these applications are in generating like subjective content in some ways. So like one of the most prominent applications we're seeing is using these language models to generate marketing copy. And then the other really famous one obviously is this art generation.
[00:43:10] And I was saying it's kind of funny that like if you think back to a decade ago when we were all hypothesizing about what AI was gonna do, we said, everyone always thought the place that they'll come last are for roles that are truly subjective and like art-based, right? Like everyone was like, you know, if you're a painter, like there's no way AI is gonna come for your job. And it turned out that that head prediction is like completely wrong. And I think it's wrong for two reasons. One because when you're generating something like an art image or even like a marketing slogan, you have a lot of leeway to kind of like do it in this like party trick way and there aren't real consequences for what you're delivering back, right? Like when you type a prompt into DALL-E and you say, generate me this random image, it doesn't really matter what it spits back to you. The fact that it's spitting back something relevant to your prompt, like that creates the moment that they're seeking to create. Oh, very cool. Right? But it isn't like, there aren't these stakes of, did they answer this question perfectly and find the relevant information inside a scientific document?
[00:44:13] And the same is kind of true for marketing copy, right? Like there isn't like a perfect way to generate a marketing slogan. You just kind of throw things on the wall and see what sticks. So guess what a machine's really good at doing? Throwing things at a wall and seeing what sticks, right? And then I was talking about this with my co-founder and he brought up another really interesting reason why this is happening is because the internet is filled with just subjective content. And it creates these giant training corpuses to build these models. So like the internet is literally just filled with marketing copy, right, like where you have titles to articles. So you could say, alright, here you have you all this training data already built for you where it's, here's the text of the article, here's the header. Okay. Build me a model that generates headers for articles, right? Like anybody with NLP skills could build you that model in not that long of a time with a giant training set of data to do it on. And the same goes for these images, right? Google Image, all the images across the internet have these captions and titles of what they are. Like if you Google search something, it has whatever your images will have a little like description of it. Well, there's your training data. You have all these pictures and all of the prompts. Now, you can train a model that can take in a prompt and spit out an image.
[00:45:21] So anyway, I think it's really funny that everyone thought that these, the more subjective thing, like it's gonna be the hardened like one or zero tasks that AI does first 'cause like those are all things that are automated. All the subjective things that make us so uniquely human, they'll never come for that. And then that was completely wrong.
[00:45:41] Adam Gamwell: Watch them come, you know? It was funny it was actually only recently I feel like I got duped that I realized or found out that, you know, when you're doing those like captcha phrases like, you know, you're like select the pictures of a boat. We're just training an AI.
[00:45:54] Eric Olson: That's why they're all street pictures 'cause it's training self-driving cars. That's why they try to make you pick out what the stop sign is or what the motorcycles are or what the stoplight is. It's training data for self-driving cars.
[00:46:06] Adam Gamwell: Does that count as publicly funded research, do you know?
[00:46:10] Eric Olson: There's probably an argument for that.
[00:46:12] Adam Gamwell: How do I get a cut of that, you know? Eric, thanks so much for joining me on the pod today. This has been really great. I'm super excited to have listeners check out Consensus. I'll put the link and get some folks to kind of test it out and see and get their thoughts of what it's like 'cause I'm really curious to see the human science community dive in and see what we can find in there. Again, taking some thoughts with the benefits of mindfulness. The question around death penalty I think is really interesting as well. So an inspiration for folks to kind of dive on in. But thanks so much for taking the time of talking with us today. And yeah, wish you the best of luck and hope we can keep in contact and, you know, dive on as Consensus keeps on building.
[00:46:45] Eric Olson: Absolutely. This has been a blast. Thank you so much, Adam.
[00:46:48] Adam Gamwell: Awesome, thanks.
[00:46:51] Thanks again to Eric Olson from Consensus for joining me on the podcast today. I am really excited at the possibilities of making it easier, faster, and more digestible to see what the latest scientific consensus is around all sorts of ideas. And in case you forgot, Consensus is currently free while in beta, so definitely check it out. Give it a spin and let me know what you find. I'm really curious at the new kinds of connections and possibilities that you could find. So I'd love to see what you're looking up and the different ideas that you're bringing together.
[00:47:15] Now, in fact, This Anthro Life and Consensus did a little collaboration that I'd love to share with you. You can check it out on the latest post from the TAL newsletter on Substack. The link to subscribe is in the show notes below. And as I've been mentioning for a little while now, it's been a goal of mine to do more writing and expand the TAL mindshare across mediums. So I hope you'll check it out and subscribe.
[00:47:32] And if you get something out of This Anthro Life, please take a moment to give the show a review on your podcast list of your choice, if that's an option. And share it with a friend who you know will love it, too. It's funny despite podcasting being a thing for years now, I think almost around 20 years, the best way to discover new shows is still through human connections and sharing with your friends. So I'd be honored if you find the show worthwhile to have you share it with a friend. And hey. Let me know about it. Shoot me a message at thisanthrolife@gmail or get in contact through the TAL website and maybe we'll have some new podcast love stories to share on air. Never a bad thing, right? And as always, thank you so much for joining and being a part of this conversation. The invitation remains open to pitch and submit ideas for episodes or blog posts, and that includes things I can make, that you can make, or that we can make together. So if you have an idea, let's get in touch. Much love, my friends. Check out consensus.app, give it a spin, and I cannot wait to see you next time on the next episode of This Anthro Life. I'm Adam Gamwell. We'll see you soon.
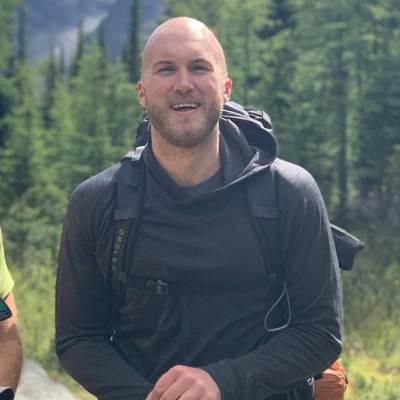
Eric Olson
Eric is a talented data science professional with a Master’s in Predictive Analytics from Northwestern University. Before Consensus, Eric worked at the sports entertainment company DraftKings, where he was an Analytics Specialist building predictive machine learning models to understand the user base better.
Eric came up with the idea for Consensus after spending years as a die-hard amateur science consumer and wanting access to better evidence-based information at the click of a button.
Eric formerly was a three-year starter for the Northwestern University Football team, is an avid skier, loves the outdoors, and hopes to convince NFL coaches someday never to punt again.